Anyone who tried to build an AI model knows that there are still significant barriers to successful AI deployment. In five steps, learn how to launch AI with greater confidence.
5 Tips for Handling the Biggest AI Obstacles
You may have heard the stat: over 80% of artificial intelligence (AI) projects never make it to deployment. While we expect to see that number decrease in the coming years, in the meantime companies face very real, and common, barriers to success. Lack of high-quality data, insufficient technical expertise, misalignment within the organization: all of these are examples of significant challenges faced by companies pursuing AI.The good news is that more and more companies are figuring it out, finding ways to make AI work for their business and launching solutions at scale. Companies building AI should learn from these successes. With the right infrastructure in place and the right tools at your disposal, you’ll maximize your chances at beating the AI odds.
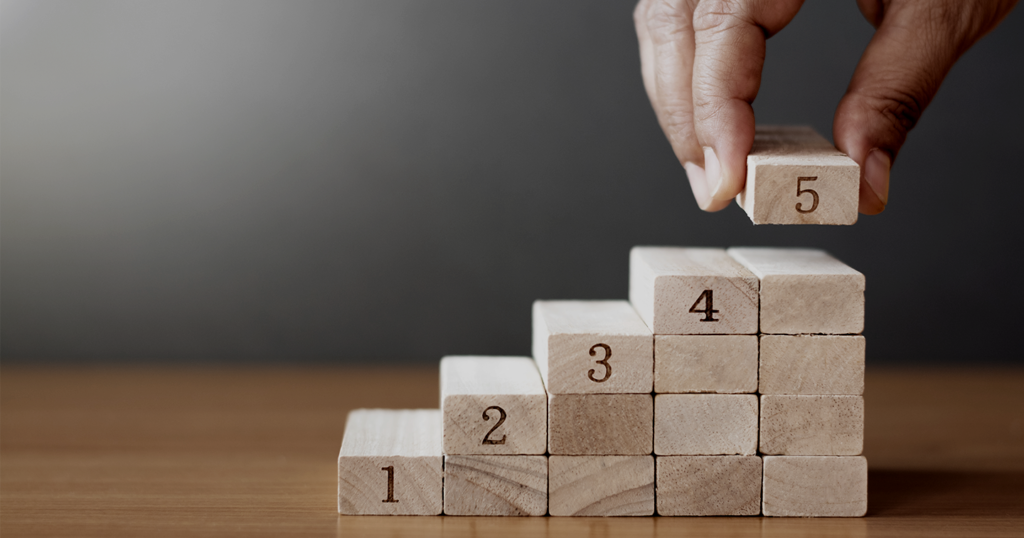
A 5-Step Plan for Addressing Challenges in AI
While not an exhaustive list of every step in the model-build process, the following are five key pieces of advice for teams looking to launch high-performing AI models.
1. Select the Right Problem and Evaluate ROI
AI practitioners often struggle from the very start of a project because they’ve unknowingly selected the wrong business problem to solve using AI. The problem might be too broad to solve effectively, ultimately too expensive, or simply not require AI for solving. Avoiding this hurdle means doing your due diligence upfront.Experts recommend executing a discovery phase, where both business and technical teams work together to select a problem, define a solution, take measure of readily-available data for that problem, and strategize on where you’ll source additional data from. In this phase, evaluate the potential ROI from multiple perspectives: whether this endeavor is essential to the business, and what to expect from success (is it cost-savings? Additional revenue?).You may realize that the data just isn’t there, and that you’ll need to find another solution that isn’t AI-based, or so data-heavy. Or you may discover that you selected a problem that will be unlikely to give you the returns you require. Any number of relevant findings can arise during the discovery process, so it’s important to clarify as much as possible at this stage.
2. Bridge the Gap Between Business and Technical Leaders
Despite progress in recent years, there are still gaps in understanding between business leaders and technical leaders when it comes to deploying AI. It’s crucial, though, that all stakeholders in your organization understand what AI can do and what its limitations are. AI isn’t bulletproof, after all; there will be uncertainty involved and there will likely be mistakes made along the way.Set expectations ahead of time so that all relevant stakeholders, including business leaders and C-suite executives, understand what the AI initiative is set to accomplish and the steps required to get there. For example, business leaders need to understand the iterative nature of building AI; models will need continuous data for testing and training purposes, and this will require added costs as the project progresses. Alignment is needed on the entirety of the project process and its associated resource requirements.If you’re deploying AI for the first time, it can be especially helpful to select a business problem and AI solution where you can easily communicate its value to business stakeholders; that way, they’re much more likely to approve the allocation of the required resources.
3. Align Technical Teams
Within the technical sector of organizations, there’s often still misalignment between groups like DevOps, IT, and data scientists. Often, these teams operate in silos; IT, for example, isn’t generally very familiar with the ins and outs of AI. For an AI project, however, all of these groups play an important role. A concerted effort must be made to connect these silos before pursuing AI.Some companies create alignment by combining various technical groups into one dedicated team that solves challenges together. This team will only function, of course, with the full support of your organization.
4. Invest in Data
Engage in data-driven development, which means investing in data quality above all else. It’s clear that model performance is most heavily correlated with data quality, more so than model complexity or other factors. You’ll need data even after deployment, as your model experiences changes in the real-world data it encounters. Investing in data (and ensuring all stakeholders understand the importance of this) will factor heavily into your success.Collecting and preparing training data is also the most time-consuming step in the model-build process. In the collection phase, you may need to source data from multiple sources, partner with a data provider, or create synthetic data to fulfill all use cases. In the data preparation phase, you’ll need to clean your data and annotate it accurately. If the latter requires the usage of subject matter experts, have a plan in place for sourcing those annotators or working with a data provider who can collect and annotate the data.Because of the massive effort required to create high-quality data, this is one area where you should build automation when possible. Create pipelines to continuously clean, annotate, and monitor your data. If needed, leverage open source tools that let you define your specific data protocols.
5. Be Prepared to Iterate
Building AI is a highly iterative process. You may need to cycle through several models, for instance, before you find the best one for your solution. You’ll need to test your model repeatedly under new data, hyperparameters, features, and other variables before reaching deployment. Even after deployment, your model will likely encounter new and unforeseen data in production and require new training. Incorporate comprehensive data pipelines to continuously retrain your model on new data.Ultimately, you should expect the unexpected. New use cases could appear in production that your team hadn’t thought about, or customers could interact with your solution differently than you imagined. In any case, be sure to have appropriate metrics in place to track model performance on a variety of KPIs. Also incorporate a feedback loop so you can understand how your customers feel about your product, and make adjustments as necessary.
Building to Scale
Many of the challenges in AI deployment come down to low-quality data, insufficient resources, and internal misalignment. If you’re able to address these barriers through strong infrastructure and upfront planning that involves all relevant stakeholders, you’ll be in excellent shape to achieve success. Completing these steps will also help you scale your AI solution to other business units, further justifying the investment of resources and paving the way for future AI projects.