AI Data Quality: Best Practices for Precise, Accurate, and Data-Centric AI
AI data quality is a key differentiator as models grow in complexity and precision. But what is high-quality data and how do you measure it? Enable your model to continuously improve with an emphasis on data-centric AI development through a combination of the right tools, AI expertise, and data sources.
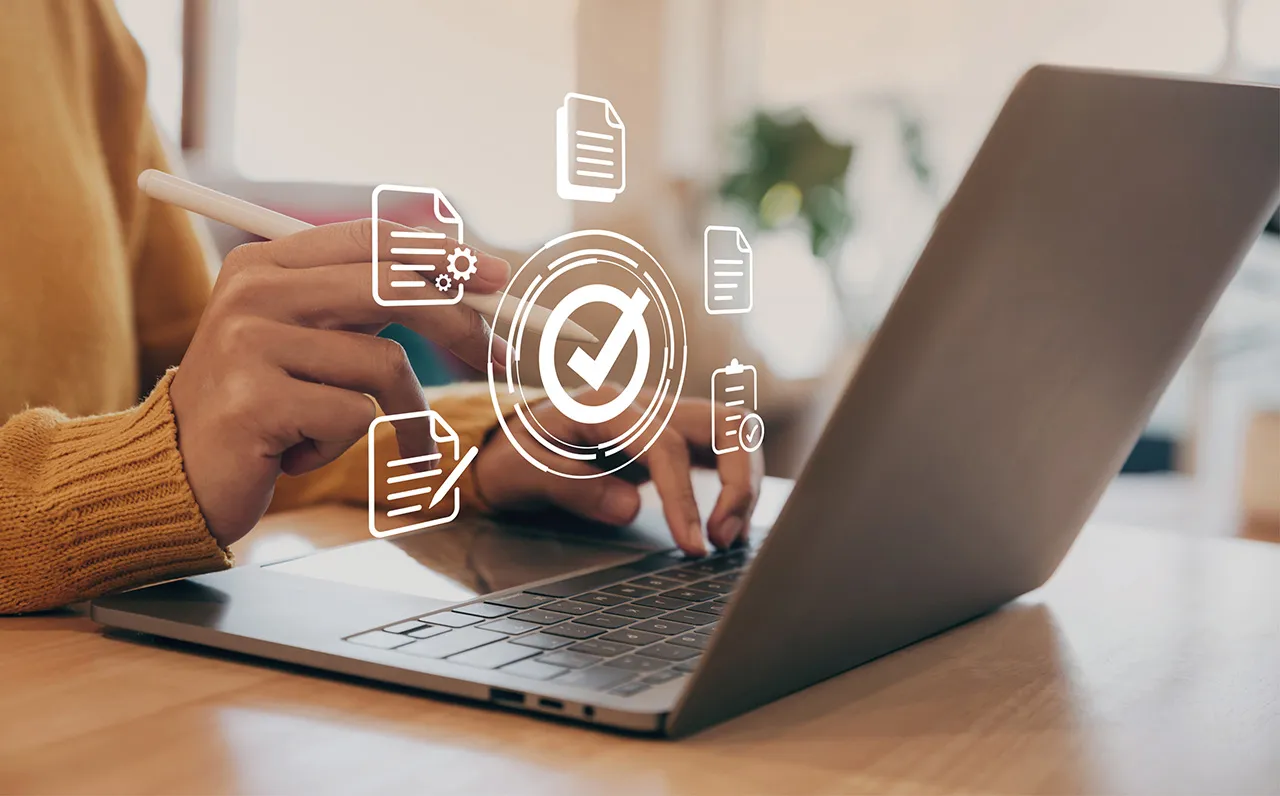
How to Measure AI Data Quality
Aligning data with model objectives is essential to ensure AI systems are built on a foundation of relevant, accurate data. Appen establishes a high foundational level of AI data quality with proprietary assets and unique analytics capabilities that define and measure data quality in a scalable way.
Precision
Ensuring data points are consistently labeled or annotated correctly minimizes variation in how similar data is treated. Techniques to enhance precision include thorough guidelines for annotators, quality control audits, and continuous feedback loops to improve labeling consistency.
Accuracy
Accuracy is critical across all AI applications from computer vision to natural language processing (NLP), as inaccuracies can directly impair model performance. Methods to maintain high accuracy involve rigorous verification steps, root cause analysis for errors, and periodic evaluations against established benchmarks.
Completeness
Minimize gaps or missing information in your datasets to cover all necessary aspects of the domain. Completeness can be enhanced by systematically identifying data gaps, ensuring representation across different categories, and integrating diverse sources to better support multimodal AI applications.
AI Data Quality Best Practices
Achieving high standards for AI data quality requires a multi-faceted approach to address the complexities of data annotation, evaluation, and risk management. Data quality best practices for ensuring reliable, scalable data quality in AI projects include:
Continuous Evaluation and Quality Monitoring
- Implement consistent evaluation practices to track data accuracy, detect quality degradation, and ensure alignment with project objectives.
- Utilize tools like Appen’s Model Mate and Quality Reporting Framework for label-level reporting and error trend analysis, providing detailed insights into data quality.
- Standardize scoring and reporting systems to evaluate contributor, auditor, and market-level performance, using root cause analysis to pinpoint and address areas of improvement.
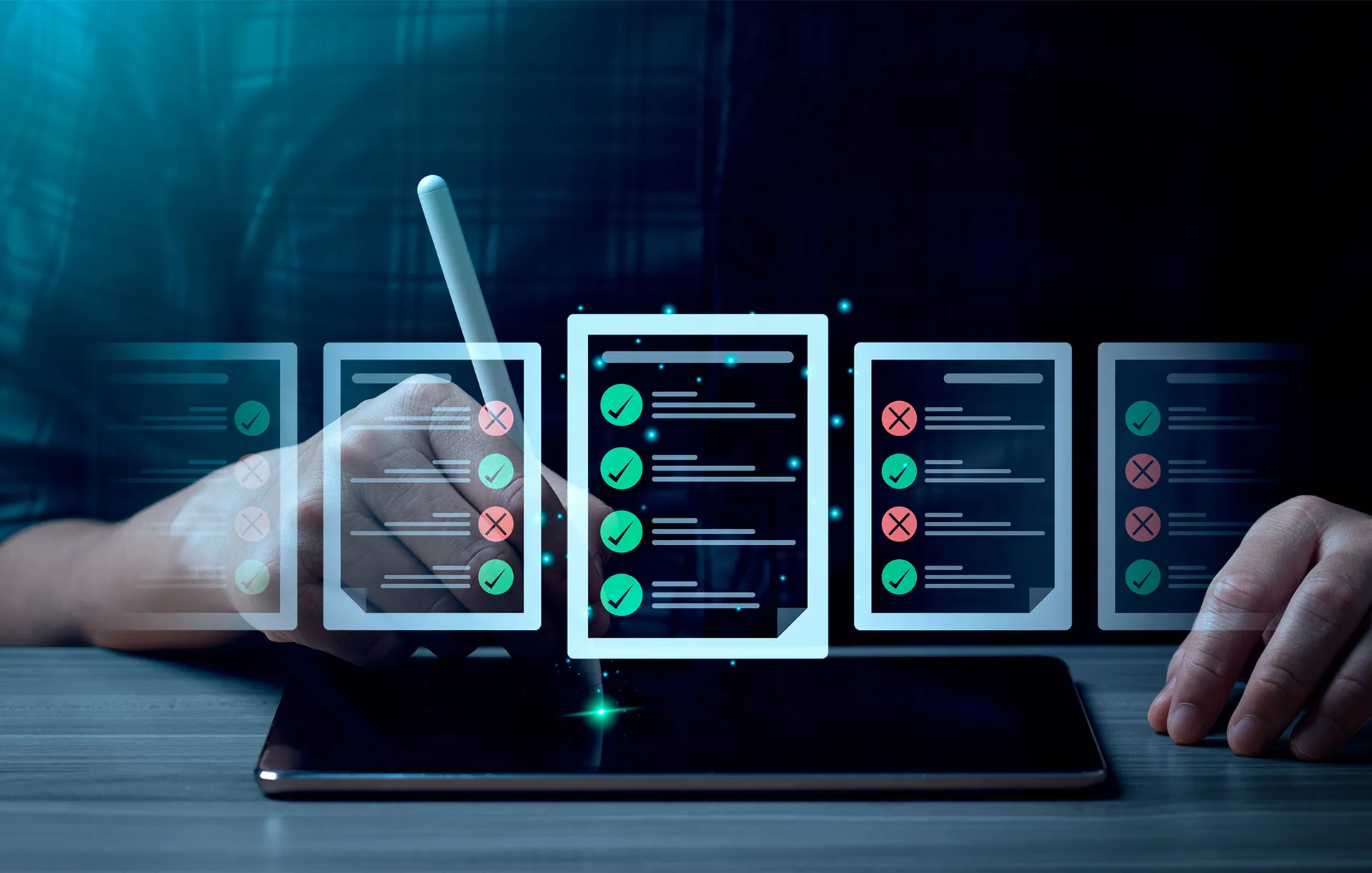
Managing Consistency, Subjectivity, and Complex Data
- Leverage techniques like Inter-Rater Agreements (IRAs) to ensure label consistency across contributors, particularly for complex or subjective tasks.
- Apply metrics such as rankings and Likert scales to measure agreement and identify discrepancies.
- Manage the variability of free-text responses by applying quality control checks on syntax and semantics, while leveraging LLMs as a judge for qualitative assessments.

Upskilling, Collaboration, and Risk Management
- Enhance contributor performance through targeted upskilling based on error trend analysis and the identification of specific skill gaps. Provide training and resources tailored to individual, market, or project needs.
- Foster proactive collaboration between stakeholders, including partners and vendors, to ensure clear and consistent guidelines. Open communication channels help align project goals and mitigate ambiguity in labeling tasks.
- Implement robust risk management strategies, such as Appen’s quality risk register, to monitor potential issues and mitigate risks. Feedback mechanisms at individual and market levels, along with client input, ensure continuous improvement and alignment with project objectives.
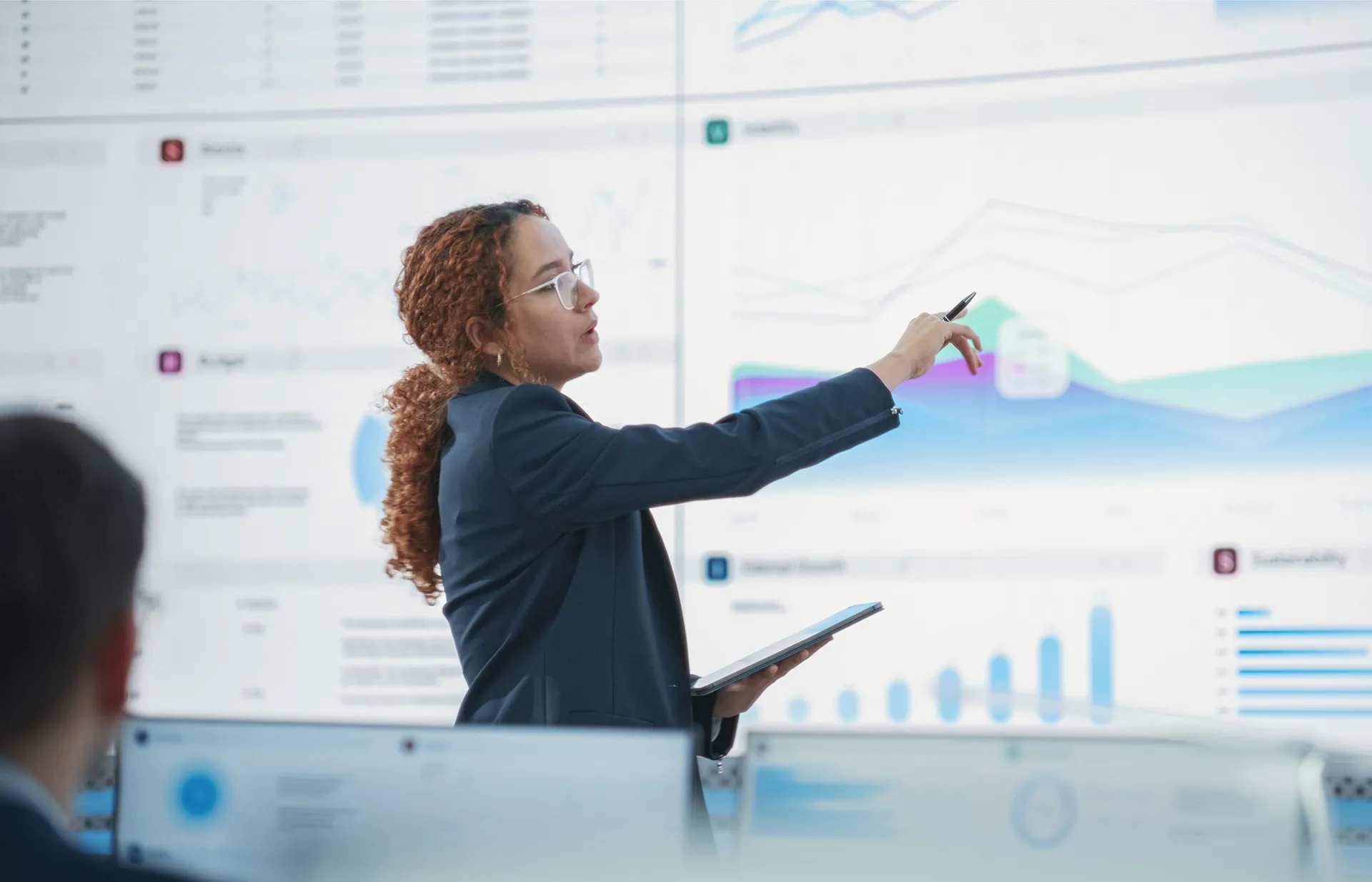
Techniques to Improve Data Quality
There are a variety of techniques available to improve your AI data quality. Maximize the impact of your data with techniques like:
Accuracy Metrics
Leverage data accuracy and efficacy metrics, such as F1 scores, to manage imbalanced datasets and prioritize critical errors.
Likert Scales
Quantify agreement levels among annotators, providing a structured approach to evaluate consistency in subjective tasks.
Inter-Rater Agreement (IRA)
Measure consistency among contributors, using metrics such as rankings to standardize evaluation across teams.
Free Text Responses
Manage variability in syntax, semantics, and relevance, ensuring consistency in open-ended data with quality control processes.
LLM as a Judge
Leverage LLMs for a scalable solution to bias mitigation and qualitative assessments of ambiguous or subjective labeling tasks.
Model Mate and Quality Reporting Frameworks
Track label-level metrics, analyze error trends, and generate tailored reports to guide improvements in data quality.
The Shift Towards Data-Centric AI
Data-centric AI is redefining how artificial intelligence systems are developed, placing a stronger emphasis on the quality and relevance of data rather than solely focusing on algorithmic advancements. High-quality data is critical to unlocking the full potential of generative AI, as it enables models to perform more effectively and adapt to real-world scenarios. This approach ensures AI systems are not only robust but also more ethical and scalable, reducing the need for continuous algorithm refinement.

Companies like Appen are driving this shift by prioritizing data quality and innovation. As highlighted in the 2024 State of AI Report, Appen’s tools and methodologies help organizations improve their data pipelines with high-quality AI data collection, tailored quality metrics, risk management, and granular reporting. By adopting a data-centric approach, businesses can achieve more accurate, efficient, and impactful AI applications that meet the growing demands of a data-driven world.
Make data your differentiator
High-quality AI data is dependent upon human expertise to deliver the data models need to continuously expand and evolve. Collaborating with an experienced AI data partner enables you to leverage their experienced project managers, linguists, and contributors to reliably generate high quality datasets at scale. By adopting data-centric practices and focusing on precision, accuracy, and completeness, organizations can build more robust and reliable AI systems.