AI Computer Vision: Unlock the Visual World
Computer vision technology continues to evolve, with new advancements in deep learning, neural networks, and AI leading to even more powerful applications. As businesses and industries increasingly adopt AI solutions, computer vision is essential for automating tasks, improving efficiency, and enhancing decision-making.
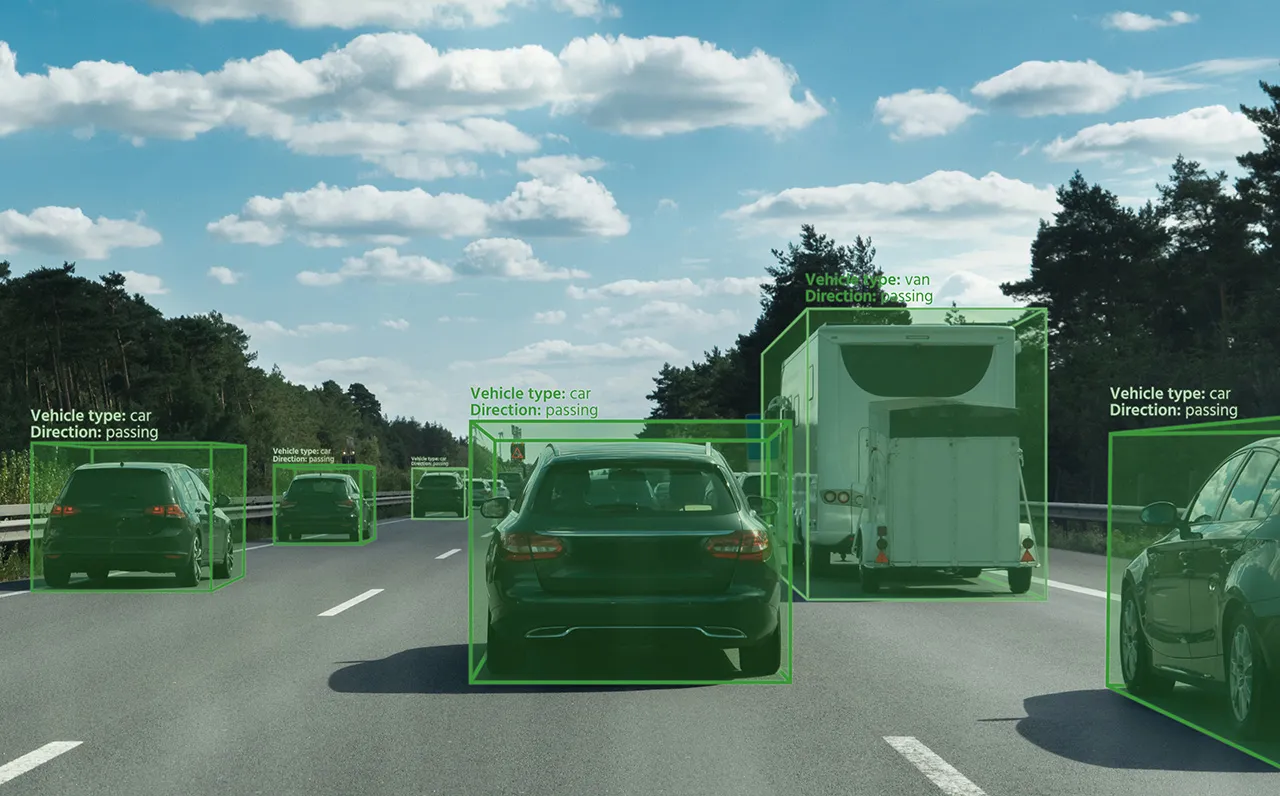
What is Computer Vision?
Computer vision is an advanced field of artificial intelligence (AI) that enables machines to interpret and understand the visual world. Leveraging machine learning algorithms, computer vision technology allows AI systems to process, analyze, and make decisions based on visual data, such as images and videos. AI computer vision plays a vital role in various AI applications across industries such as recognizing objects, facial expressions, and even medical anomalies.
What is Computer Vision Used For?
Computer vision has numerous applications across different industries, helping businesses automate processes and improve decision-making. Some key computer vision applications include:
Retail and E-commerce
Visual search and product recommendation engines improve customer experience by identifying products from customer images and preferences.
Creative Expression
Computer vision empowers users to express their creativity with visual effects like social media filters, video game avatars, and AI-generated media.
Autonomous Vehicles
AI computer vision is innovating transportation, enabling vehicles to detect and interpret road signs, pedestrians, and obstacles, ensuring safe navigation.
Security
AI-powered surveillance systems use computer vision to identify individuals from large databases, improving safety and streamlining access control in both corporate and public settings.

How Computer Vision Technology is Combating Wildfires
A global security and aerospace company partnered with Appen for the high-quality data annotation and model evaluation they needed to predict wildfire paths, considering complex factors like terrain, wind, and real-time fire location. The collaboration pushed the boundaries of what's possible in wildfire prediction and response.
Key AI Computer Vision Tasks
Computer vision technology addresses a variety of tasks essential for automating and analyzing visual data. Some of the most important computer vision tasks include:
Recognition
Computer vision models can identify and extract specific information from images – such as faces and text – to detect key elements within visual data. Essential computer vision recognition tasks include:
- Optical Character Recognition (OCR): Extracting and converting text from images or scanned documents into machine-readable text, often used in document digitization or license plate recognition.
- Facial Recognition: Analyzing and identifying faces in images or videos for security and authentication purposes.

Classification
Computer vision technology enables automatic recognition, interpretation, and organization of visual data in images and videos. Examples of classification in action include:
- Image Classification: Categorizing images into predefined classes based on their content, such as identifying whether a picture contains a dog, car, or tree.
- Object Detection: Identifying and classifying specific objects within an image or video, such as pedestrians, vehicles, or animals.
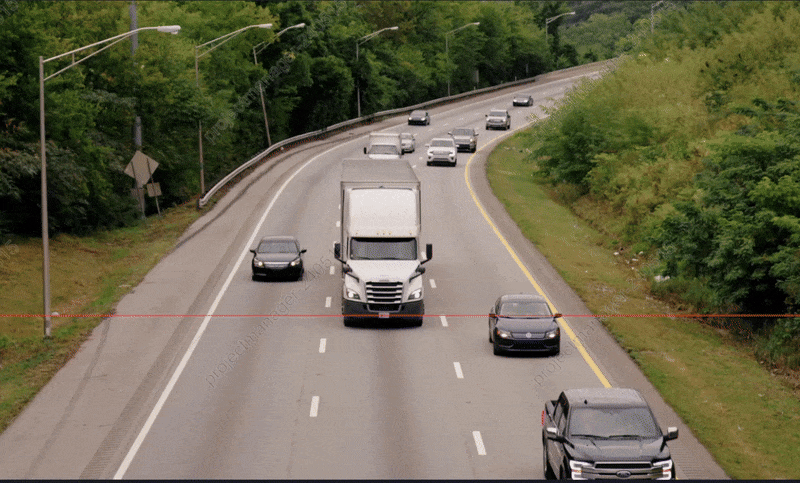
Comprehension
Applications such as video summarization and captioning require advanced computer vision models capable of interpreting and analyzing multiple modalities of data such as audio and written text. Comprehension is vital for tasks such as:
- Semantic Segmentation: Dividing an image into segments and labeling each part, such as distinguishing between focal objects and background features.
- Media Summarization: Identifying the contents of an image or video and interpreting that data to generate descriptions or captions.

Generation
With the rapid growth of LLMs, generative AI models now create images and videos from natural language prompts. These models must first comprehend visual data to produce realistic media, making this task dependent upon the others for success. Computer vision enables tasks such as:
- Image Generation: Creating new images for creative purposes as well as business use cases such as generating e-commerce images.
- Video Generation: Generating dynamic visual media such as animations and synthetic videos for individual and business use cases such as social media.

Reconstruction
Fields like augmented reality (AR), virtual reality (VR), and robotics require models to leverage advanced spatial awareness to comprehend multi-dimensional data. Examples of reconstruction tasks include:
- 3D Modeling: Generating three-dimensional models from two-dimensional data, such as converting a blueprint into a 3D rendering.
- Historical Preservation: Leveraging AI and 3D modeling to recreate ancient artifacts and sites in realistic renderings.
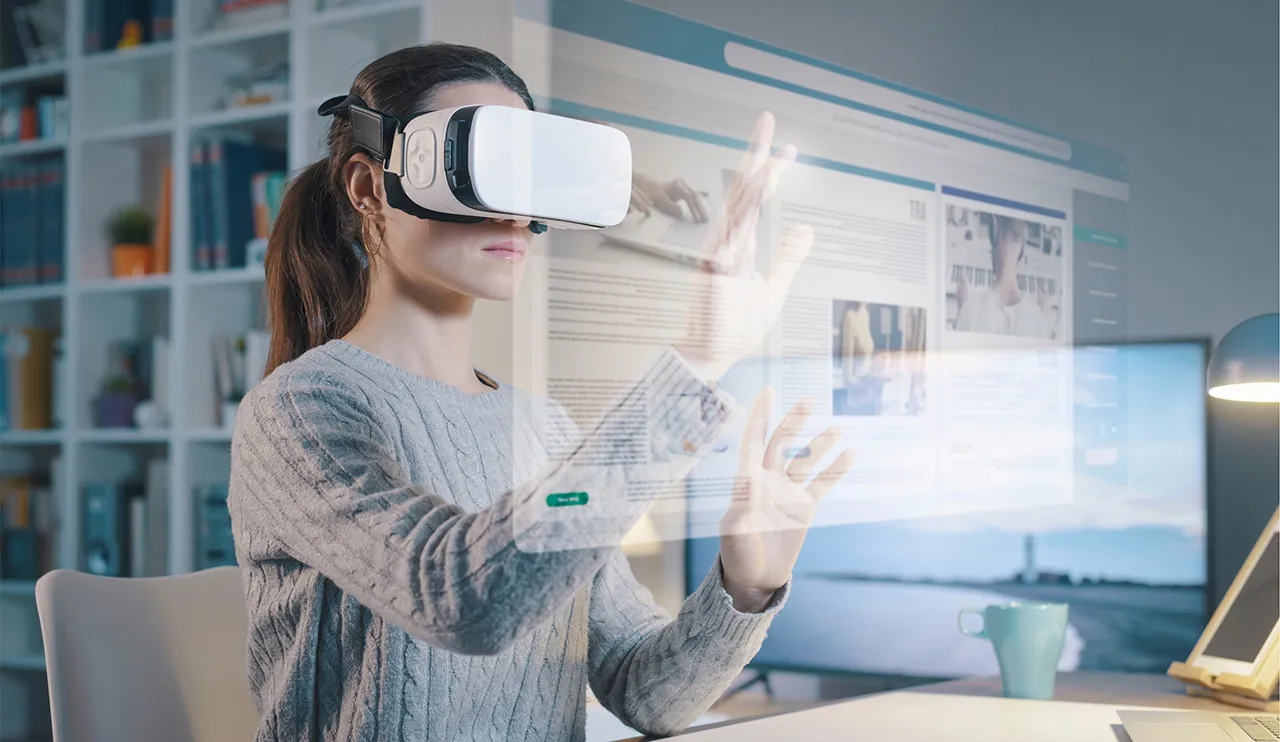
How to develop a computer vision model
Building a robust computer vision model requires a structured approach, combining high-quality data, model development, and continuous refinement. The process typically follows three key phases.
Data Collection
High-quality, diverse datasets are essential to ensure the foundation model is fine-tuned for real-world environments. Images, videos, and sensor data must be gathered from various sources and scenarios to provide the model with comprehensive information. For example, training computer vision models for security requires broad spectrum of video and image data across diverse weather and lighting conditions.
Data Annotation
For supervised learning, the collected data must be accurately annotated and labeled to create a high-quality computer vision dataset. This involves tagging objects, people, and other relevant features in the visual data. Precise annotation is essential for teaching the AI how to understand and categorize data. By correctly labeling objects, faces, or actions within a dataset, you provide the model with reliable information that allows it to recognize similar patterns in new data.
Evaluation & Fine-Tuning
After the model is trained using the annotated data, its performance must be continuously evaluated and fine-tuned. Regular evaluation is crucial to ensure your computer vision model makes accurate predictions and decisions based on new visual inputs. Fine-tuning improves model accuracy, reduces errors, and enhances its ability to generalize across different tasks and environments. It is important to keep in mind that model development is an iterative process, and you will likely need to repeat data collection, annotation, and model refinement to continue improving your model over time.
The Future is Multimodal
Modern computer vision applications, such as autonomous vehicles, require models to process and integrate various types of input data, including video, audio, and images simultaneously. Multimodal AI enhances traditional computer vision models by enabling them to analyze and interpret richer, more complex information. By processing multiple data streams and types, multimodal AI models are capable of deeper comprehension and create richer outputs. By combining computer vision with other data modalities, multimodal AI is paving the way for more advanced, versatile AI systems.
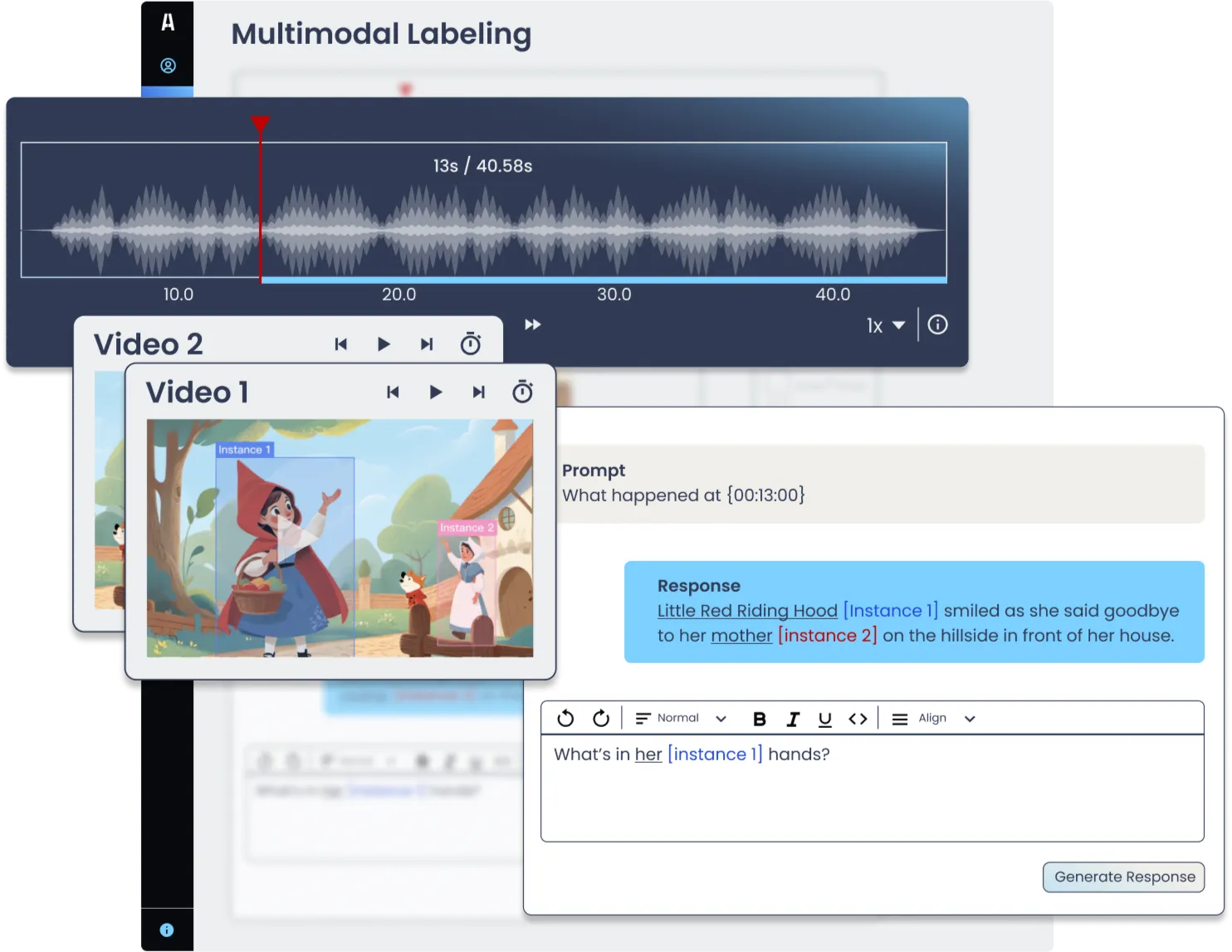
Why Appen?
Appen supports leading computer vision AI companies by providing comprehensive data collection, annotation, and model evaluation services. We offer tailored solutions to help you train and optimize your AI models, ensuring they perform accurately in the real world. We support your AI computer vision projects with services such as:
Customized Data Collection
Gather and curate data tailored to your specific use case, ensuring that your models are trained on the most relevant and representative datasets.
Off-the-Shelf Datasets
Leverage pre-existing datasets to quickly train your model. Appen has prepared computer vision datasets including thousands of images for facial recognition, object detection, and document processing.
Expert Data Annotation
Our team of experts uses advanced tools and human-in-the-loop processes to label and annotate data accurately, helping your models learn from clean, reliable inputs.
Ongoing Model Evaluation
Continuously monitor and evaluate your computer vision models, testing their performance and making necessary adjustments to ensure optimal accuracy in real-world applications.
Ready to Innovate?
Investing in high-quality data and evaluation is the best way to maximize your computer vision investment. Appen’s expert will give you a competitive edge in leveraging the full potential of computer vision AI.