Generative AI: Transforming Creativity Across Industries
Generative AI gives machines the power to create in revolutionary ways – reshaping industries from art to business with the ability to generate new texts, images, videos, and more from a simple prompt.

What is GenAI?
Generative AI (GenAI) is a branch of artificial intelligence that produces new data based on patterns in existing datasets – such as leveraging large language models (LLMs) to create written text, imagery, and videos. Foundation models in generative AI, such as GPT-4 and Llama-3, power these capabilities, automating creative processes, assisting in complex problem-solving, and enabling a wide range of practical applications across various industries.
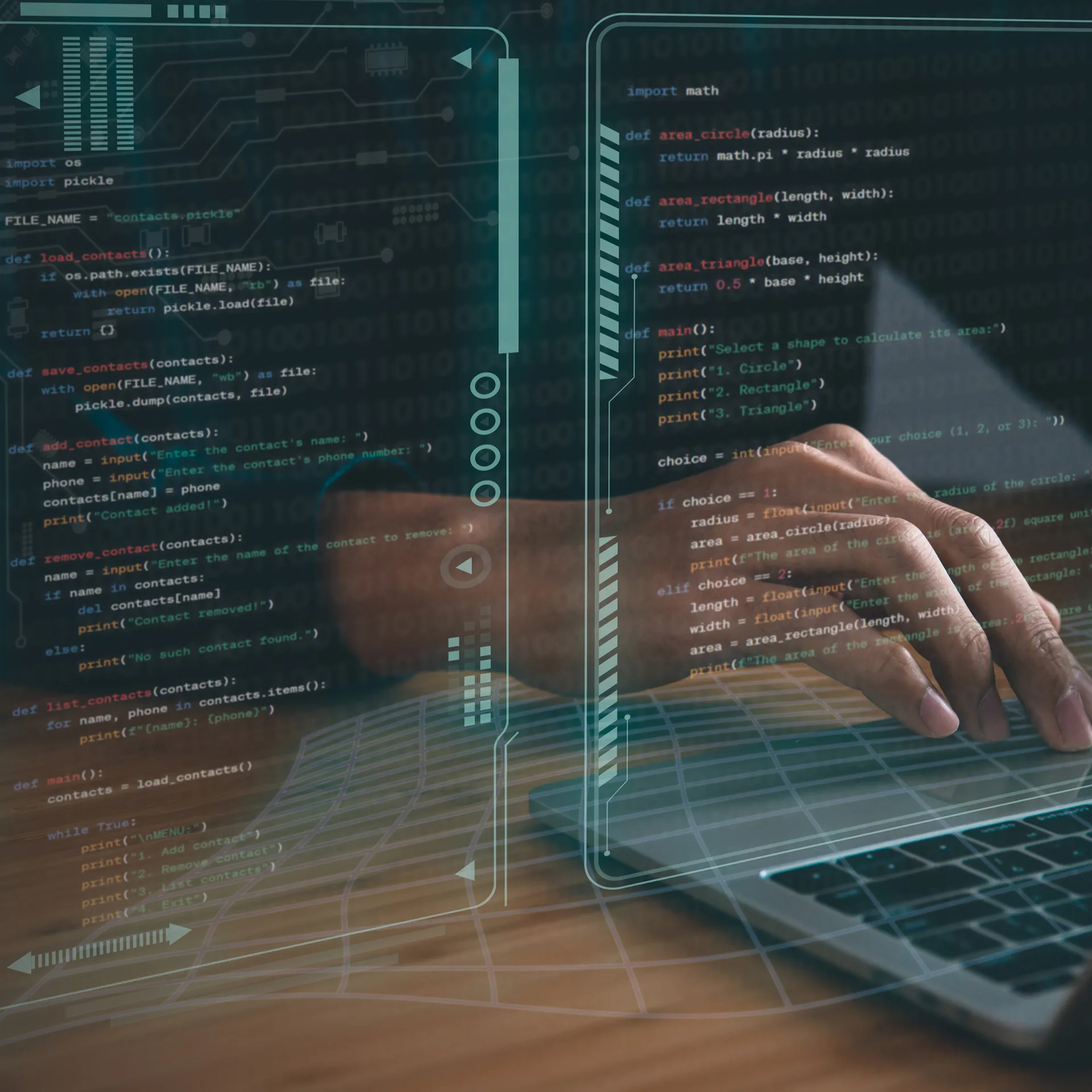
How Does GenAI Work?
Generative AI leverages natural language processing and complex deep learning models trained on extensive datasets to develop powerful tools. The two core GenAI architectures are:
Generative Adversarial Networks (GANs)
Transformer Models
Key Applications of GenAI
Generative AI platforms are transforming businesses by enabling new content creation and automation capabilities – such as powering Text-to-Image tools for visual assets, Text-to-Speech for accessibility, and Image-to-Text for ecommerce and content automation.
GenAI in Action
Generative AI can be leveraged in numerous ways across industries ranging from social media to manufacturing. GenAI platforms are available to support general use cases such as video generation as well as niche applications such as music generation.
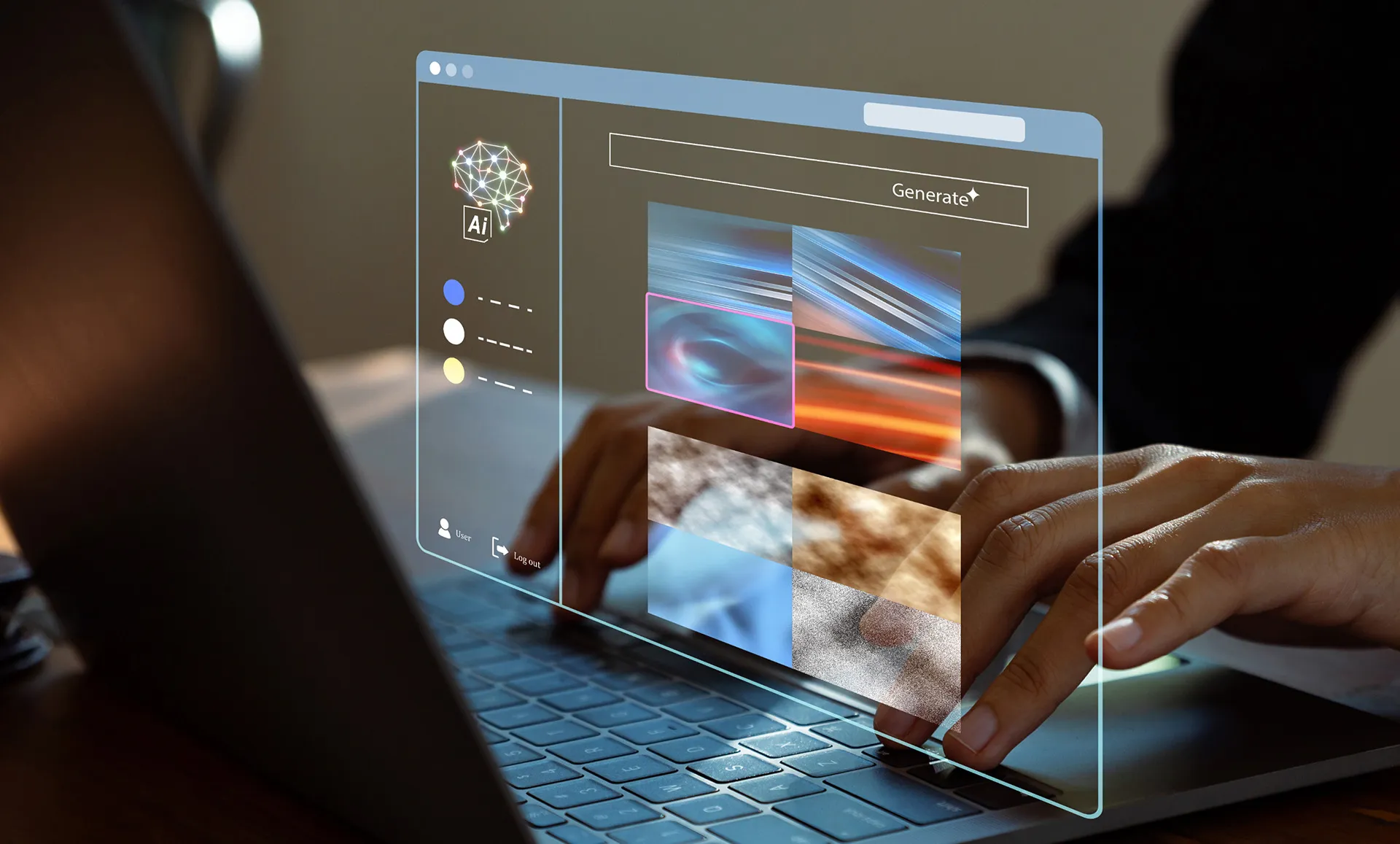
Leverage Generative AI solutions such as:
Meta Audiocraft
Generate custom music and audio tracks for advertisements, brand campaigns, or immersive customer experiences.
OpenAI Sora
Streamline content creation with AI-powered video generation and marketing materials.
Jasper.ai
Write blog posts, ad copy, or social media captions efficiently at scale with an AI-driven content creation platform.
Character.ai
Create conversational AI personalities for customer support, training simulations, or brand engagement.
What Challenges Does Generative AI Face with Respect to Data?
Despite its potential, generative AI faces significant data-related challenges that impact its effectiveness and reliability. Data-centric AI principles play a crucial role in improving the quality of datasets used to train these models, ensuring accurate and reliable outputs. Here are the primary issues:
Data Quality and Bias
AI data quality is one of the key challenges faced by GenAI. Without high-quality, unbiased data, GenAI outputs can reflect unintended biases and increase liability risk to end users.
Data Privacy
Given the large volumes of data needed, generative AI must address data privacy and compliance regulations, particularly in fields like healthcare and finance.
Scalability
GenAI requires substantial computational resources to process and generate content, which can be cost-prohibitive for smaller organizations.
Integration Across Modalities
Combining inputs from various data sources, as seen in multimodal AI, can present challenges in data synchronization and consistency.
Benefits of GenAI
Generative AI improves efficiency, creativity, and personalization, especially in ecommerce, content creation, and customer service. Key benefits include:
Increased Efficiency
Automate everyday tasks like content generation, programming, and meeting minutes with generative AI, ultimately saving time and resources.
Enhanced Creativity
Utilize GenAI as a creative collaborator, generating new ideas in fields such as writing, design, and marketing.
Customization
Adapt existing information for new use cases such as generating recaps for videos and meetings, converting technical documentation into accessible summaries, and transforming notes into a PowerPoint slide.
Generative AI Platforms for Businesses
Generative AI platforms are increasingly crucial for businesses, providing tools and solutions that drive content automation, personalization, and advanced analytics. These platforms incorporate foundational GenAI models and often leverage RAG capabilities to enhance customer experiences.
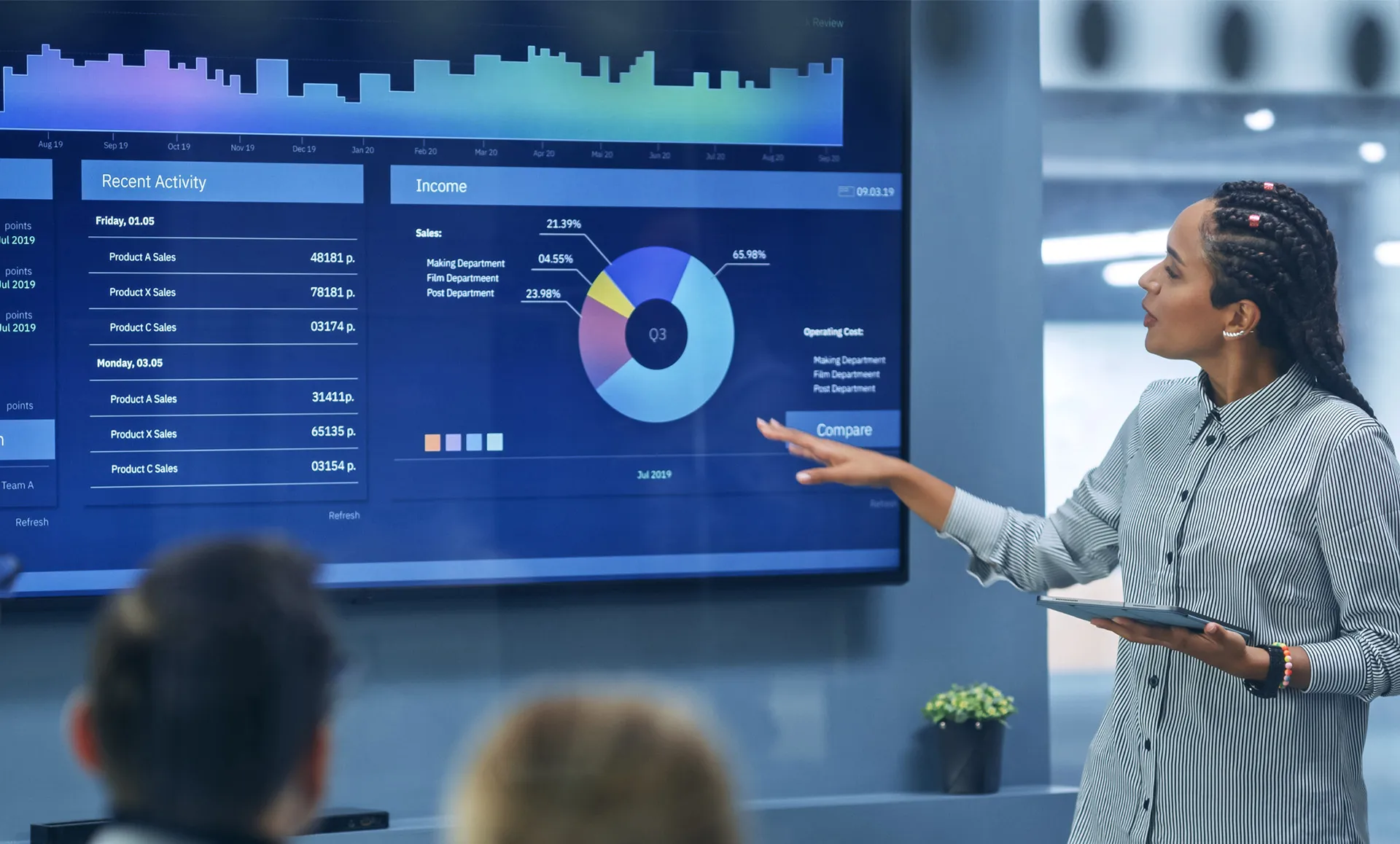
How to Implement Generative AI in Your Business
To adopt generative AI successfully, businesses should focus on AI data quality, strong infrastructure, and specialized expertise:
Data Quality Evaluation
Conduct thorough assessments and clean existing datasets to ensure they are accurate, relevant, and diverse enough to support robust model performance.
Use of Data Augmentation Tools
Leverage tools to generate additional datasets with varied inputs, improving the model’s ability to generalize across diverse scenarios.
Collaboration with Experts
Work with AI professionals to design and implement solutions tailored to your industry and specific business needs.
Scalable Infrastructure
Invest in infrastructure capable of supporting the extensive computational demands of training and deploying generative AI models effectively.
Knowledge Management
Apply multimodal AI, such as computer vision, to extract, classify, and summarize information from visual data, enhancing decision-making and collaboration.
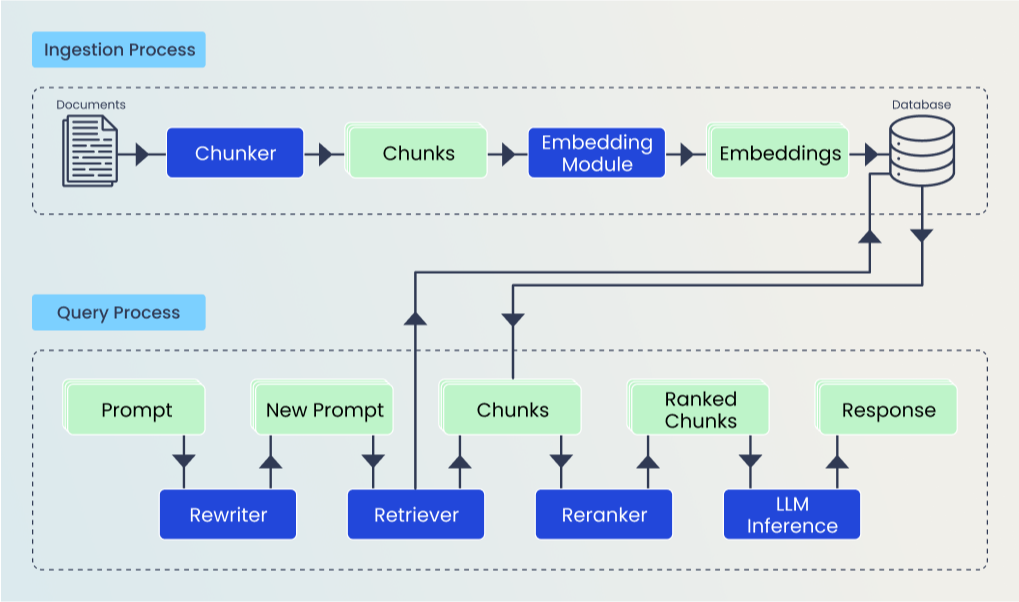
What is RAG in GenAI?
Retrieval-Augmented Generation (RAG) is a technique in GenAI that enhances output accuracy by combining retrieval mechanisms with generative models. RAG generative AI models draw on external datasets, often in real time, to supplement their own knowledge, making responses more informed and relevant. RAG systems are especially useful in industries where real-time data, such as customer interactions or stock levels, can improve personalization and decision-making.
The Future of Generative AI
The future of generative AI will see continued improvements in model accuracy, efficiency, and ethical standards. As GenAI technology rapidly advances, multimodal AI models will become increasingly adept at integrating diverse data types such as text, images, and audio. These advancements will enable more seamless and intuitive interactions, significantly enhancing user experiences across industries. By addressing challenges like data quality, bias, and scalability, while maintaining high ethical standards, generative AI is poised to drive a new era of innovation, personalization, and practical intelligence.