Build business-centric AI more efficiently and ethically with these words of advice from experts in the field.
The following is adapted from Real World AI.It’s becoming increasingly clear that AI is the future for business. AI models can save time, increase efficiency, and drive revenue. As more and more businesses adopt AI, it soon won’t be a nice-to-have, but a must-have in order to compete in the marketplace.As a business decision-maker, that can be overwhelming. Unless you have a strong tech background, there’s probably a lot you don’t understand about AI. It can be tempting to hire a tech team and have them handle everything to do with AI.That’s a mistake. For AI to be effective, it must be business-centric, and that means you, as a business decision-maker, play a key role in AI development. Here are three things you must do to help create better AI for your business.
#1: Avoid Organizational Telephone
Too often, an AI project idea makes its way from conception to implementation through a game of organizational telephone. The path from the original business stakeholder who dreams it up to the machine learning engineer or data scientist who will actually build it inevitably passes through five steps and three management changes.Just as in childhood games of telephone, a lot is lost in the process. Brooke Wenig, Machine Learning Practice Lead at Databricks, had an experience that exemplifies organizational telephone games and the kind of damage they can cause.While serving an internship at MyFitnessPal, an app that allows users to track their diet and exercise, Brooke was given the task of building a model to classify the foods users entered into the app into groups, such as fruits, vegetables, etc.Brooke spent more than six weeks cleaning the data and creating mappings into a clear hierarchy, only to find out the groupings she had created were not at all what the product team needed or wanted. So, she had to start over from square one.Time and resources had been lost because no one had told her which groups the app could actually monetize, and the groups she had created were worthless from a business standpoint. The reason Brooke didn’t have the information she needed was the business folks—the product team—had not been in close contact with the person executing the AI model, and because of this, the project completely stalled for weeks on end.A lot more than just data science goes into an AI model’s creation—business decisions make up the most significant portions of the work involved. So as a business decision-maker, you must get involved granularly, to ensure that the people building the AI model are doing so in a way that is additive to the business.
#2: Pick the Right Objectives, Success Measurements, and Milestones
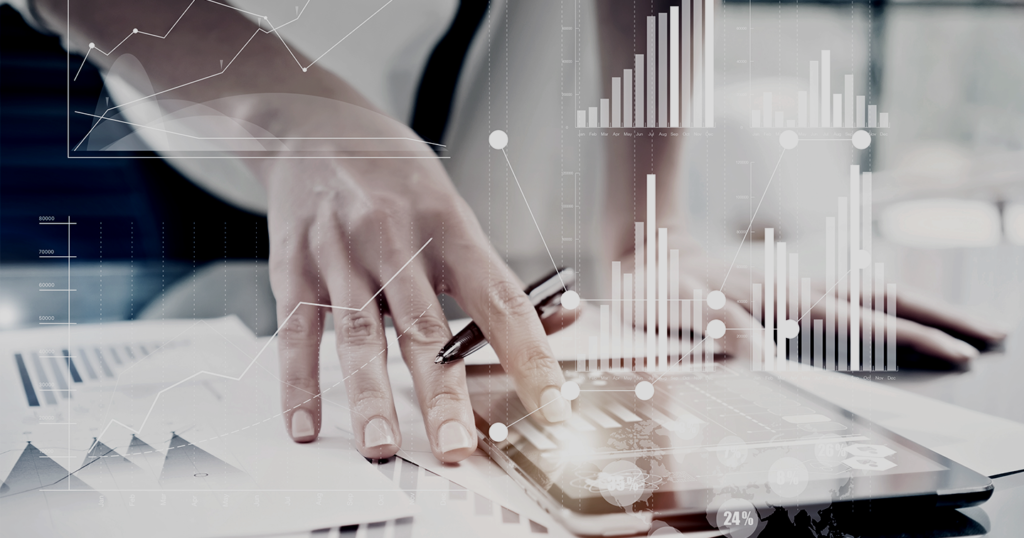
It’s not uncommon—in fact, it’s shockingly common, even among businesses seasoned in AI work—that a huge investment of money and resources is made in an AI solution, only to have it produce the wrong result or even fail completely. A company can do all the “right” things, hire smart and experienced people, and have the best intentions, and still wind up expensively spinning their wheels rather than achieving objectives.Case in point: in October of 2013, IBM announced an exciting partnership with the University of Texas’s MD Anderson Cancer Center. The alliance would use the cognitive computing system of IBM’s supercomputer Watson to take cancer research to new heights. After more than three years of work and more than $62 million spent by MD Anderson, the project was put on hold, having never actually used the technology on a patient.One of the reasons was that the MD Anderson team incorrectly identified and failed to deeply understand the specific problem that AI had the potential to impact. Applying machine learning to an objective as broad as “cure cancer” will quickly reveal the limitations of the technology. An AI model needs a specific problem to solve in the present, not a faraway future goal.AI requires constant maintenance, management, and course-correction to continue to provide meaningful, desired output. Without having a clear idea of the objectives, success measurements, carefully defined expectations, milestones, and guidelines when embarking on a project, the chances of success are slim—as MD Anderson discovered.While you might not understand the intricacies of building an AI model, you do understand what it takes to successfully manage a project. By helping to establish the right objectives, success measurements, and milestones, you can ensure your company’s AI model stays on the right track.
#3: Consider the Ethical Implications of Your AI Model’s Decisions
AI can have a real impact on people’s lives, so the way models are designed matters. It’s important that your company’s AI usage drives business results, but you also must consider the ethical implications of your AI model’s decisions.For instance, if you’ve decided to optimize your model to the point where it’s 90 percent accurate, that means that 10 percent of the time, it’s getting the decision wrong. If your model is classifying support tickets as higher or lower priority, maybe that 10 percent isn’t such a big deal. But if it’s identifying reports of sexual assault, misclassifying 10 percent could represent a significant ethical failure, not to mention the introduction of substantial liability.If you’re introducing a model that used to do something that relied on humans making ethical decisions, you’ll want to guard against your data science team being too homogeneous. The job of the data scientist is essentially to encode intentional bias into the model to make a decision; that’s how decisions are made. If the humans supplying the data and creating the algorithms introducing that bias don’t consider enough context on sensitive areas that usually rely on human judgment, they could introduce unintentional bias.Microsoft’s Twitter chatbot, Tay, is a perfect example. In essence, Tay was supposed to learn how to converse with humans through Twitter by studying the user-generated dialogues tweeted at her. Unfortunately, there was no filter on exactly what Tay could or would learn from fellow Twitter users. As a result, Tay was fed a million malicious conversation examples by 4chan. It ended up becoming an extremist chatbot that promoted Nazism.Again, while you’re not the one building the model, you play an important role here. You must communicate ethical priorities and ensure your data science team has diverse viewpoints in order to prevent unintentional bias.
Building Business-Centric AI
AI isn’t magic. It’s a collection of technologies that can be harnessed to make decisions that serve a set of goals. People make AI, and people train AI systems with data collected and prepared by people.Your data science team understands the technological aspects of AI, but you are the one who understands the people and business aspects of it. By eliminating organizational telephone; picking the right objectives, success measurements, and milestones; and considering the ethical implications, you can help ensure that your business’s AI is more successful and will serve your users better.For more advice on building smart, responsible, business-centric AI, you can find Real World AI on Amazon.Alyssa Rochwerger is a customer-driven product leader dedicated to building products that solve hard problems for real people. She delights in bringing products to market that make a positive impact for customers. Her experience in scaling products from concept to large-scale ROI has been proven at both startups and large enterprises alike. She has held numerous product leadership roles for machine learning organizations. She served as VP of product for Figure Eight (acquired by Appen), VP of AI and data at Appen, and director of product at IBM Watson. She recently left the space to pursue her dream of using technology to improve healthcare. Currently, she serves as director of product at Blue Shield of California, where she is happily surrounded by lots of data, many hard problems, and nothing but opportunities to make a positive impact. She is thrilled to pursue the mission of providing access to high-quality, affordable healthcare that is worthy of our families and friends. Alyssa was born and raised in San Francisco, California, and holds a BA in American studies from Trinity College. When she is not geeking out on data and technology, she can be found hiking, cooking, and dining at “off the beaten path” restaurants with her family.Wilson Pang joined Appen in November 2018 as CTO and is responsible for the company’s products and technology. Wilson has over nineteen years’ experience in software engineering and data science. Prior to joining Appen, Wilson was chief data officer of Ctrip in China, the second-largest online travel agency company in the world, where he led data engineers, analysts, data product managers, and scientists to improve user experience and increase operational efficiency that grew the business. Before that, he was senior director of engineering at eBay in California and provided leadership in various domains, including data service and solutions, search science, marketing technology, and billing systems. He worked as an architect at IBM prior to eBay, building technology solutions for various clients. Wilson obtained his master’s and bachelor’s degrees in electrical engineering from Zhejiang University in China.