Understanding natural language is one of the great aspirations of artificial intelligence. While solving it could have exciting implications for society, it’s going to take an unprecedented volume of training data to make it happen. This is especially true in the interpretation of health data from a seemingly infinite array of sources — and a big reason why we’re so excited to see CrowdTruth.org leveraging CrowdFlower, now Appen, to train IBM Watson.Watson represents the state of the art in computational linguistics and computer vision put into action. It uses its never-before-seen understanding of language and imagery to comb through volumous datasets to unearth helpful information and make predictions (such as tips for disease diagnosis). According to Lora Aroyo, Principal Investigator of CrowdTruth, Watson acts as “a cognitive prosthetic to extend the decision making capabilities of an expert,” such as a doctor, who will utilize it as a tool for recommendations on how best to analyze a patient’s condition.In parallel, data enrichment platforms have become a valuable resource for data scientists looking to automate and scale the cleaning, data labeling, and enrichment of data using human intelligence for machine learning — ie., training data creation. While Watson continuously engages in active learning, its intelligence is strengthened by the quality of the training data it takes in from crowd contributors on data enrichment platforms such as Appen.

Watson achieved noteriety when it won Jeaopardy a few years back. Image via Atomic Taco
What’s next for data enrichment and Watson?
Lora Aroyo from VU University Amsterdam, Chris Welty from the IBM Watson Research Center and Robert-Jan Sips of IBM Netherlands are leading the charge with CrowdTruth. Their work focuses on the labeling of training data that combines both subject matter experts and crowd contributors to strengthen Watson’s machine learning algorithms. What they’ve discovered is groundbreaking.
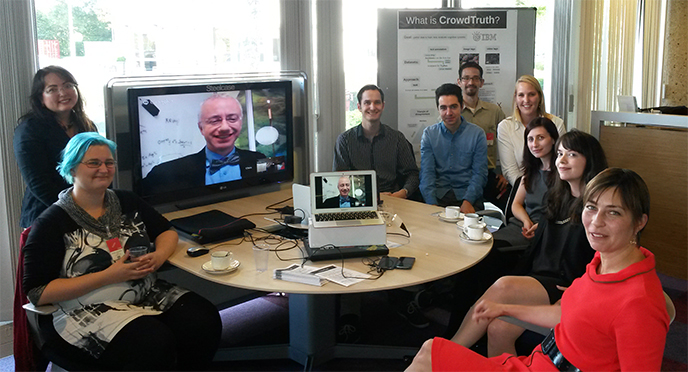
The CrowdTruth TeamCrowdTruth has found that expert annotators, highly paid health professionals working on training data creation, agree only 30% of the time. While the “popular crowd vote” covers 95% of expert agreement. Why? Experts don’t pay close attention to granularity in linguistic expression. Crowd contributors do. What this means, is that the collective intelligence of an unbiased crowd is as good, if not better, than expensive experts sitting in a room. The diversity of their annotations help Watson understand the details that expert trainers gloss over.I recently spoke with Lora Aroyo, who summarized CrowdTruth’s approach to training data creation:“The crowd of anonymous workers, typically unbiased from possible domain expertise, is processing text examples from a pure linguistic perspective, and in this way captures the diversity of interpretations provided by expert annotators.”In years past, the only path to clean training data was a laborious grant process and onboarding an army of contractors which was costly, time consuming and far from scalable. But today, researchers can simply switch Appen’s massive, on-demand workforce on-and-off to structure and extract knowledge from vast quantities of medical texts, images and videos. So much so, that CrowdTruth is proving that these layman data labelers are more effective at interpreting semantic content than paid professionals. The reason being, is that experts interpret data with pre-formed biases. Wheras crowd contributors, especially if you’re asking a bunch of them per data point, as CrowdTruth is, interpret data in a way that accounts for the spectrum of possible interpretations. Thus, Watson ingests a broader and more reliable vector to draw from as it computes predicitons.CrowdTruth’s training data complements medical expert inputs with the primary purpose of extracting insights from medical documents. By introducing people-powered data enrichment into Watson’s machine learning workflow, the system can supplement the need for expert trainers and more rapidly extract crucial knowledge from the likes of Wikipedia articles, patient case reports and beyond.
What Are the Implications of the CrowdTruth Research?
This framework will contribute to Watson’s ability to perform medical text analysis that promises to advance clinical research and the personalization of medical care in unprecedented ways.Imagine Watson being able to interpret a patient’s current symptoms and help prescribe the right treatment at blazingly fast speeds. How will do it? By synthesizing a doctor’s inputs alongside an automated analysis of a patient’s decades-old medical record, seasonal flu data for the area, and the universe of other medical knowledge amassed in Watson’s brain. What does that mean? Sick people are treated faster and better.On the clinical side, pharmaceutical companies can accelerate the development of drugs and researchers can dive deeper into their study of disease and genetics. In short, better medicine and more efficient clinical outcomes.By combining the expertise of medical professionals with the power of artificial intelligence, we very well could see these advances realized in our lifetimes. It’s an exciting prospect and we’re thrilled Appen is at the bleeding edge.
Dig Deeper:
If you’d like to dig deeper into CrowdTruth, check out the slideshow below, take a look at their GitHub, browse CrowdTruth’s team papers and presentations or take some time to read The Three Sides of CrowdTruth, that was recently published in the inaugural issue of the Journal of Human Computation.