Financial Service Providers Should Plan for AI Roadblocks
An influx of artificial intelligence (AI) investment in the financial services space has brought forward new questions around data security and transparency. These, among other challenges of AI in financial services, are particularly essential to address as data management practices evolve with the introduction of new AI solutions. Organizations must be aware of expected challenges as described below and build safeguards to keep forward momentum.
Security and Compliance
One of the main challenges of AI in financial services is the amount of data collected that contains sensitive and confidential information requires additional security measures to be implemented. The right data partner will offer a variety of security options, have strong data protection with certifications and regulations, security standards to ensure your customer data is appropriately handled. Look for data partners compliant with industry-specific or region-specific data regulations, such as SOC2 Type II, HIPAA, GDPR, and CCPA. As well as offer options such as secure data access (critical for PII and PHI), secure annotation and onsite service options, private cloud deployment, on-premise deployment, and SAML-based single sign-on.
Localization
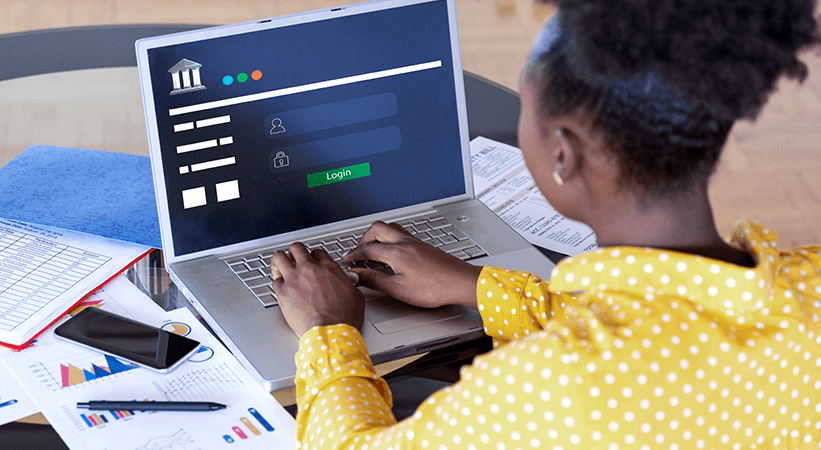
Localization is especially vital within the financial services industry. Because financial companies often need to design models with the multiple markets they serve in mind, it’s essential to factor in the challenges of AI in financial services across different languages, cultures, and demographics to properly customize the customer experience.Localization projects are great for your data partner to support you with as they can leverage teams of skilled linguists to develop things like style guides and voice personas (formal, chatty, etc.) and optimizing across many languages. It’s great that your model knows how to understand English, but do you have a plan to expand to Spanish, Korean, or Japanese? What about the regional specifics of each customer base?In some instances, off-the-shelf datasets can be incredibly helpful with expanding your model in new markets.
Transparency, Explainability, and Trust
Creating AI models that provide accurate predictions will only be successful if explained to, understood by, and trusted by customers. Because customer information is likely being used to develop these models, they’ll want to be sure that their personal information is being collected responsibly, handled, and stored securely. Some will even want to understand the basics of how it’s being used.While the most advanced AI applications are more challenging to explain, you can always go back to the training data you used to develop the model and extract some explainability from the data structure, inputs, and outputs. The validation and retraining processes can shed more light on how your models make predictions and please your customers.
Siloed Data
Building the future is complicated enough with the above challenges of AI in financial services, and that doesn't even factor in data pipelines. Connecting several dozen different data pipeline components and integrating a plethora of APIs on top of security and compliance concerns to leverage siloed data is no easy feat. In order for a financial institution to do this effectively, they need to ensure their available data is correctly collected and structured and that the information enables machine learning models to predict according to the business goals set out in the AI program.To bring pilots to production, turn to a data partner with extensive security offerings for support. Their expertise will enable financial service companies to put together these demanding components for the consumer experience, paving the way for success and scale.
Looking Ahead
When it comes to launching world-class AI, the opportunity for the financial services industry is massive – whether you’re working to improve conversational AI experiences with a chatbot and site search or build models to support your customer support agents. It’s evident that only a fully operational model that reaches deployment will deliver any kind of business value – and the best way to beat the less-encouraging odds is to safeguard against identifiable challenges and select use cases where reliable training data (with the right data partner) can get you there.While the path to entirely AI-driven automation for financial services is gradual, we’re confident that more and more organizations will need substantial amounts of reliable training data to get their AI projects into the real-world. Success is only possible by ensuring data is handled securely and with transparency and by stepping away from siloed approaches. Teams that will achieve the most significant ROI will mitigate potential roadblocks before deployment and focus on an exemplary data management strategy.__Download our ebook, AI Solutions in Financial Services, to learn more.