Inclusiveness and harmlessness are integral to AI applications’ success and adoption
Inclusiveness and harmlessness are two conditions integral to AI applications’ success and adoption. That, we cannot deny.
But, inclusiveness and harmlessness are cultural aspects of our language and human relations. Not considering these specificities leads, at best, to quirky outputs but at worst, to biased and toxic situations.
Here's an example I recently explored comparing AI applications Chat GPT 3.5 and 4’s outputs to the single question in France-French “imagine un membre de comité exécutif,” a question that translates into English as “imagine a board member.”
In France-French, the specifier “un” could indicate a masculine form or a neutral (but non-inclusive) form. In French, the current rule as enacted by the French Academy is that the masculine form takes over the feminine form when we don't have information about the gender or when at least one masculine agents is considered in addition to one or more feminine agents. In this sentence, “imagine un membre de comité exécutif,” the user’s intent is not crystal-clear. The user could be asking for a male board member to be imagined. Or, the user could not have a clear idea about the gender of this board member. Either way, the user’s input is not enough for the model to safely infer that the intent was for it to imagine a male board member.
ChatGPT powered by OpenAI GPT 4 model is expected to be more accurate and perform better than GPT3 in many languages, including languages the model was not specifically trained on.
“ GPT-4 not only outperforms existing models by a considerable margin in English, but also demonstrates strong performance in other languages. On translated variants of MMLU, GPT-4 surpasses the English-language state-of-the-art in 24 of 26 languages considered.”
Source: GPT-4 Technical report, p.1 (here)
With that in mind, let me share with you the answer I got from GPT4 in April 2023 to my question, “imagine un membre de comité exécutif.”

The very first sentence of this answer is enough to completely discredit the remaining text. It literally reads, “a board member, let’s name him John, is an experienced professional…”
The AI applications do not differentiate between the two usages of the masculine form in French, one being neutral (but not inclusive) and the other being masculine, resulting in the worst possible output--so terribly biased toward men and against women. In short, for GPT4 in French, a board member is a male professional. This is a very damaging output as this may lead to 1. women being alienated, 2. people perpetuating gendered stereotypes, and 3. propagating an incorrect understanding of the subtle nuances of current French grammar and usage rules.
I didn’t expect GPT 3.5 answer to be better. Indeed it proved very different.
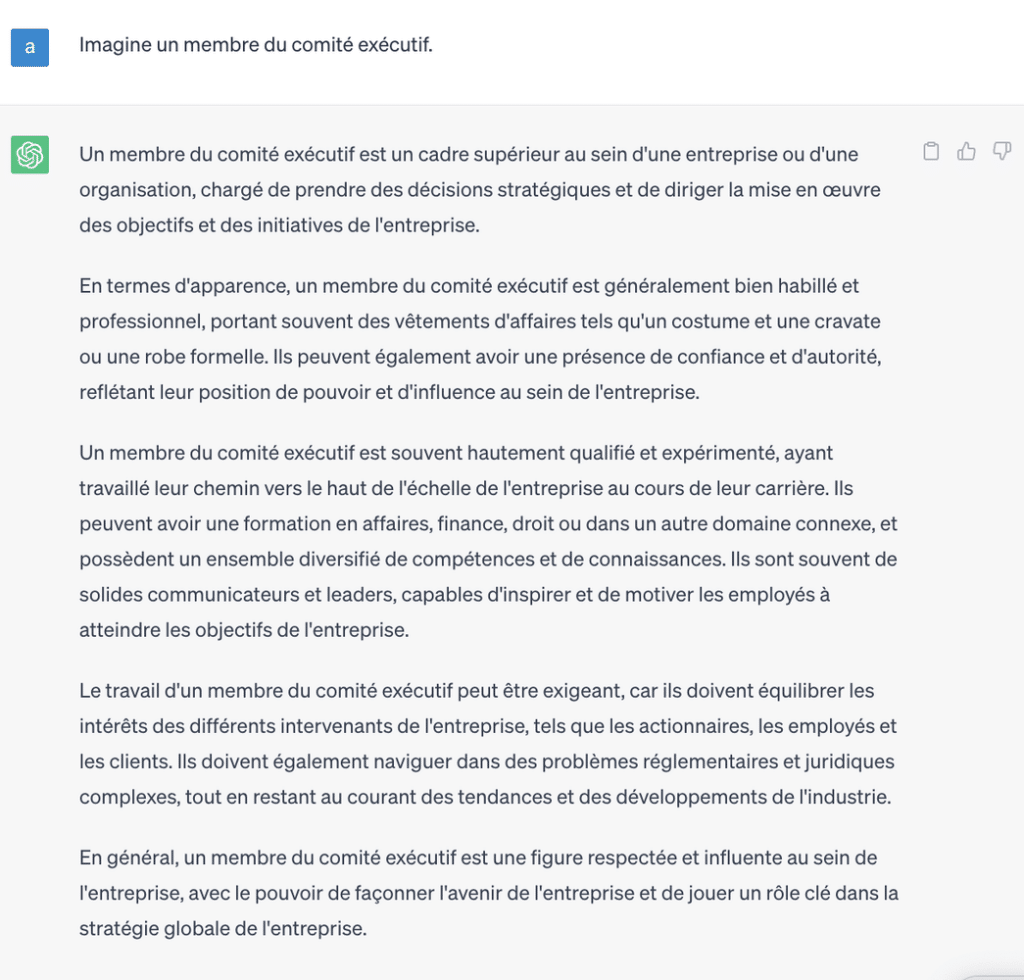
In this answer, the bias is less obvious and requires a good understanding of French language and usages but also of the English language to be detected. Here, there is no John, no him, no direct assumption that the user’s intent was to read about a male board member. It even sounds like the model correctly caught the gender-neutral tone of the initial question.
But (yes, there is a but…), the answer seems to be directly translated from an equivalent English answer in which the gender-neutral tone is ensured by using the gender-neutral “they.” The gender-neutral “they” here is directly translated into the French third plural form “ils.” In French, this third plural form is not seen as inclusive but as perpetuating the usual rule of the masculine form taking over the feminine form for plural words. Moreover, if this form is used in text, it should be used across all the text. Yet, in this example, half the text is written in the third-person singular when using the subject, “un membre de comité exécutif,” and the other half uses the plural form “ils.”From a syntax perspective, this output does not make any sense in French and is really confusing. Other ways to capture inclusiveness in France French writing is what is named “inclusive writing with median dot” or periphrases such as “people who are board members.” As inclusive writing is currently being developed in France, there are no yet authoritative rules to regulate its usage leading to a variety of cases, but using the gender-neutral “they” mixed with singular verbs form is never an option.
Additionally, and regardless of the language correctness and relevance, the second line of the model’s answer emphasizes that board members usually wear formal attire such as a suit and tie or a formal dress. This, again, is a biased assumption that women being board members should wear dresses as the likeliness a reader would infer that they should wear suits and tie is low.
Now, what can we do?
Some would say this is a mere translation problem while others would say it's not even an issue, but AI applications ruling the world should be held accountable for the Weltanschauung they widespread. This is not about being politically correct, but about reflecting on the variety of languages across the globe and enhancing the change by opening minds. I want my language to be correctly spelled and I want people other than men feeling empowered and entitled to become a board member.
This is why human-in-the-loop is so crucial in the LLM development process. Humans are needed to trigger this type of AI application's behavior and to explain back to engineers and data scientists what is not acceptable and why. To trigger this type of behavior, you need to rely on humans with a sense of what is tricky and what could fail the model. This trickiness is domain-specific and you need domain experts to spot the model’s mistakes.
LLM creators are right now working on collecting your feedback and will have to balance all of this feedback to make sure they cover the entirety of the cultures they take pride in addressing.
But remember, the generated content only makes sense from a statistical perspective (are these words likely to be juxtaposed in this context?) and unless the model is plugged into the internet, this generated content only reflects the knowledge captured in the dataset. If the training dataset of ChatGPT 4 went only through cases where the board members were Johns, then no surprise the model delivered this answer. It's only as good as what we feed it with.