How to Collect Data Efficiently and Responsibly for Your AI Initiatives
Data collection continues to be a major bottleneck for teams building artificial intelligence (AI). The reasons vary: there may be a lack of sufficient data for a use case, new machine learning (ML) techniques like deep learning require more data, or teams don’t have the right processes in place to get the data they need. Whatever the case, there’s a growing need for accurate and scalable data solutions.
Best Practices for Collecting High-Quality Data
As an AI practitioner, developing a plan for data collection requires asking the right questions.
What kind of data do I need?
The problem you choose to solve will indicate what kind of data you need. For a speech recognition model, for example, you’ll want speech data from speakers that represent the full range of customers you expect to have. This means speech data that covers all of the languages, accents, ages, and characteristics of your target customers.
Where can I source data from?
First, understand what data you already have available internally, and whether it’s usable for the problem you’re trying to solve. If you need more data, there are many publicly-available online sources of data. You can also work with a data partner to generate data through crowdsourcing. Another option is to create synthetic data to fill in gaps in your dataset.The other element to keep in mind here is that you need a steady source of data long after launching your model to production. Make sure your data source is equipped to provide continuous data for retraining purposes post-launch.
How much data do I need?
This will depend on the problem you’re trying to solve as well as your budget, but generally the answer is: as much as possible. There’s generally no such thing as too much data when it comes to building machine learning models. You need to make sure that you have enough data to cover all of the potential use cases of your model, including edge cases.
How do I ensure my data is high-quality?
Clean your datasets before using them for training your model. This means removing irrelevant or incomplete data as a first step (and checking that you weren’t counting on that data for use case coverage). Your next step is to accurately label your data. Many companies turn to crowdsourcing for access to large numbers of annotators; the greater variety of people you have annotating your data, the more inclusive your labels will end up. If your data requires specific domain knowledge, leverage experts in that field for your labeling needs.By answering these questions, you can start to build a data pipeline that enables you to collect high-quality, accurately-labeled data efficiently. Ultimately, having a repeatable, consistent data pipeline will help you scale.
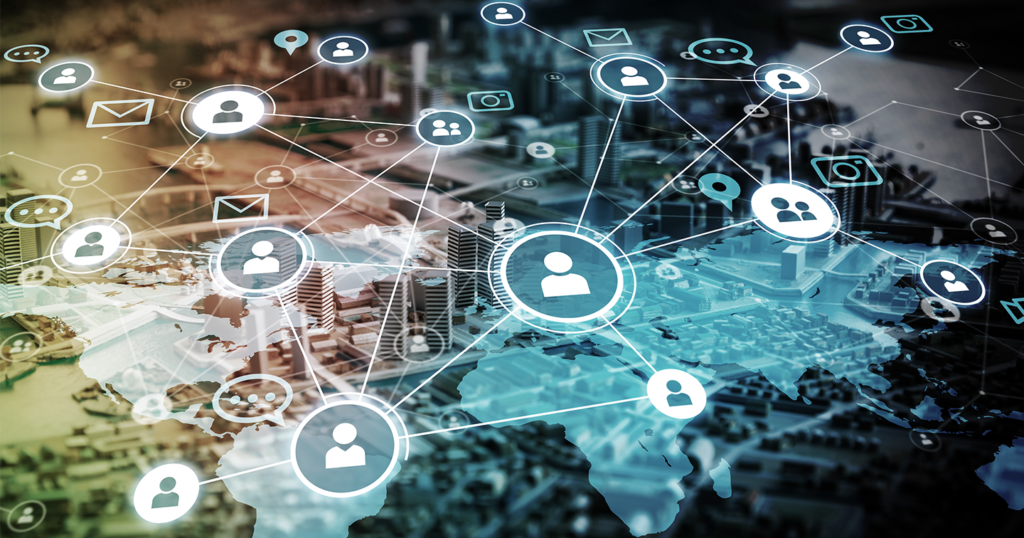
Where Responsible AI Plays a Role
You should always perform data collection with a responsible AI lens, as ethical AI starts with the data. Clean data-sourcing should be top priority, meaning you need to be obtaining your data in an ethical way. This is especially true when you’re working with secure and confidential information, such as medical records or finances. Follow data protection legislation for your region and industry and when selecting a data partner, check that they are compliant with these regulations as well. Your data partner should have security protocols in place, as should you, to ensure that customer data is treated respectfully and responsibly.
Expert Insight from David Brudenell - VP, Solutions & Advanced Research Group
Inclusivity is better than bias
Over the past 18 months at Appen, we have seen a big shift in the way that our customers are engaging with us. As AI has evolved and become more ubiquitous, it has clearly surfaced gaps in how it was built. Training data plays an important role in reducing bias in AI and we have advised our clients that creating a representative, inclusive crowd to collect data creates faster, better and more economically beneficial AI. As nearly all training data comes from data collected by people, we advise our customers on focusing on inclusivity first in the sample design first. This creates more work and experimental design, but the ROI is greatly improved as compared to a more simple sample design. Simply speaking, you get more diverse and accurate ML/AI models with more specific demographics and in the long run, this is far better than trying to ‘fill gaps’ by removing bias from your production ML/AI models.
Think of the user first
A well-designed data collection is the sum of its parts. An inclusive sample frame is the foundation, but what drives throughput and data quality is having a user-centric approach to all parts of the engagement process: invitation to the project, qualification, onboarding (including Trust & Safety) the experiment experience. Many times, teams forget that there is a human who completes these projects. If you forget this, you will have poor project uptake and data because of lower-than-average written experiments and UX.When designing your experiment and user flow, ask yourself if you would be willing to do the work. Make sure too that you always personally test the experiment from end-to-end. If you get stuck or frustrated, then there are improvements to be made.
Interlocking quotas - from six to sixty-thousand
If you take the US census and build an experiment around six data points: age, gender, state, ethnicity and mobile ownership you have over 60,000 quotas to manage?This comes from the impact of interlocking quotas. An interlocking quota is where the number of interviews/participants required in the experiment is in cells requiring more than one characteristic. Using the above US census example, there will be one cell with n-number of required users with the following characteristics: male, 55+, Wyoming, African American, Owns a 2021-generation Android smartphone. This is an extreme, low-incidence example, but by creating your own interlocking matrix before you price, write your experiment or go in-field, you can check to find very difficult or nonsensical combinations of characteristics that may impact your project’s success.
Incentives matter more than ever
Finally, and most importantly is to review the incentive that you are paying for a user to complete the experiment. Commercial trade-offs are common when designing data collection experiments, but what you must not cut is the incentive to the user. They are the most important part of the team that will produce timely, high-quality data for you. If you choose to pay less to the user, you will have slower uptake, quality and in the long-run have to pay more.If you are constrained by budget, look for advice on global purchasing power parity (PPP); can your dollar go farther in different regions in the world? Reduce your quota requirements - can you group 24-40 year olds into one group rather than two? These are just a few techniques that you could employ to get maximal commercial value for your project.
What We Can Do For You
Appen provides data collection services on our platform to improve machine learning at scale. As a global leader in our field, our clients benefit from our capability to quickly deliver large volumes of high-quality data across multiple data types, including image, video, speech, audio, and text for your specific AI program needs. We offer several data collection solutions and services to best fit your needs.Our approach to data collection starts with inclusivity. With our global, diverse crowd of annotators, we support our clients in developing data that’s representative of your customers. Operating with over 25+ years of expertise, we’ll work with you to optimize your data pipeline efficiency to its maximum.To discuss your data collection needs, contact us.