The following is adapted from Real World AI.
I joined eBay back in 2006, and in 2009, the company was in very bad shape. Its share price was at a historical low, well off its near-$24 historical high; it was cutting costs, growth was negative, market share was shrinking, and the technology team wasn’t empowered to innovate.
Put simply, the company was in serious trouble.
They turned this around, largely thanks to investing in technology. In particular, the company began the journey to use technology, data, and AI to drive business. I was lucky to join and build the search science team, which was one of the first few teams to leverage machine learning to optimize buyer experience and help them find desired items more easily on eBay’s site.
We set out to build an AI model that would improve the customer experience and drive revenue, but we didn’t get it perfect on the first try. Often, with AI, things go wrong before they go right. As a business owner or decision-maker, or as an engineer or data scientist, it’s important to understand why an AI model might not work as intended, so that you can fix it and use AI more effectively.
Building Our First Model
Because we wanted to drive revenue, when we first set out to build our AI model, our team focused on increasing purchases per session: the average number of items a buyer purchases in one user session.
With that goal in mind, our AI model emphasized the sales (how many times an item was sold) over impressions (how many times an item was viewed), and less expensive items, which sold more frequently than expensive ones, were ranked higher than other items.
We tried different machine learning models—models to rewrite buyers’ queries, models to generate features to be used in the ranking model, and models to rank the final search results. We then ran a series of A/B tests to assess the model results, with great success. Many of the models proved that buyer conversion had increased. Other teams were motivated by these successes and started to put in the effort to increase their purchases per session.
Everything looked rosy. That is, until the finance team observed that those A/B testing wins didn’t translate into increased revenue.
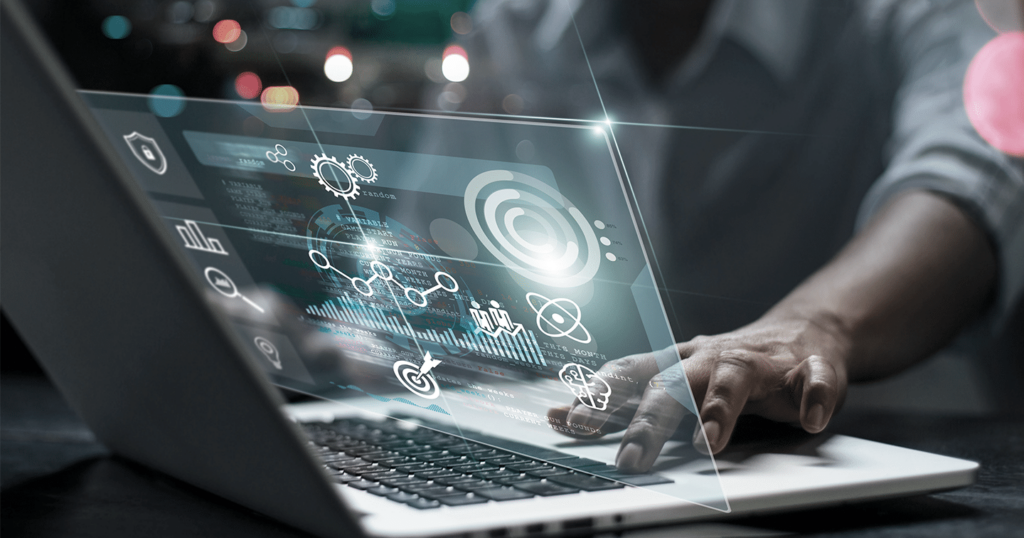
A Working Model is Not Necessarily a Profitable One
We’d gone wrong somewhere, and we needed a solution—fast. We were hurting revenue for the company at a time when it couldn’t afford to lose a single cent.
We dug deep into the search results for different queries and found one interesting phenomenon: very often, we ranked accessory items on the top. For example, many iPhone cases would rank at the top of the results when buyers searched the term “iPhone.” Although those accessories were popular on the site, they weren’t what the user had been searching for, so it created what we call “accessory pollution” and led to a bad user experience.
Aha! We had figured out why revenue had taken a dip; a $10 iPhone case represents much less revenue than a $300 iPhone. Our model was recommending the less expensive accessories when it should have been recommending the higher-priced phone.
Our model was working exactly as we had built it, but we’d built it to do the wrong thing.
Pick the Right Measurement
Success, much of the time, is all about what you choose to measure.
When we started our journey, the technology team unified different goals into one single goal focused on increasing sales. It’s a very customer-centric choice to say your only goal is to sell more—but that’s what sellers and buyers want and what we were ultimately paid to do.
After many rounds of discussions, we started with measuring the success by purchases per session. Our AI model succeeded in the goal but created a bad user experience and failed to deliver business growth. We needed to find a new solution with a different AI model, and even more importantly, a new way to measure the AI model’s success. Clearly, “purchase per session” created the wrong motivation in our AI models and our team.
The lesson was obvious: be careful to pick the right measurement because it will inform the direction of your AI.
Later on, we incorporated price-related signals to the model, which fixed “accessory pollution” problems. More importantly, we changed the measurement from purchase per session into gross merchandise value (GMV) per session. With these changes, we had not just a working model, but a profitable one.
AI Takes Work, but It’s Worth It
Once our team showed the whole company how powerful machine learning and data could be, more teams started to leverage AI as the powerhouse for business growth. This ultimately had a huge impact on revenue and helped engineer the spectacular turnaround of the company.
By 2012, eBay’s share price had increased by 65 percent, and the company had enabled about $175 billion in commerce—around 19 percent of global e-commerce and nearly 2 percent of the global retail market.
If eBay had not embraced AI, the company would likely be in a very different place right now. Today, missing the boat on AI can quite literally mean losing the competitive edge in your industry.
Tackling AI can feel overwhelming and overly technical, but it’s important to remember that it’s a process. You might not get it right on the first try, but if you learn from your mistakes—and work to measure the right things—you can build powerful tools with real impact.
For more advice on building effective, business-centric AI, you can find Real World AI on Amazon.
Alyssa Rochwerger is a customer-driven product leader dedicated to building products that solve hard problems for real people. She delights in bringing products to market that make a positive impact for customers. Her experience in scaling products from concept to large-scale ROI has been proven at both startups and large enterprises alike. She has held numerous product leadership roles for machine learning organizations. She served as VP of product for Figure Eight (acquired by Appen), VP of AI and data at Appen, and director of product at IBM Watson. She recently left the space to pursue her dream of using technology to improve healthcare. Currently, she serves as director of product at Blue Shield of California, where she is happily surrounded by lots of data, many hard problems, and nothing but opportunities to make a positive impact. She is thrilled to pursue the mission of providing access to high-quality, affordable healthcare that is worthy of our families and friends. Alyssa was born and raised in San Francisco, California, and holds a BA in American studies from Trinity College. When she is not geeking out on data and technology, she can be found hiking, cooking, and dining at “off the beaten path” restaurants with her family.
Wilson Pang joined Appen in November 2018 as CTO and is responsible for the company’s products and technology. Wilson has over nineteen years’ experience in software engineering and data science. Prior to joining Appen, Wilson was chief data officer of Ctrip in China, the second-largest online travel agency company in the world, where he led data engineers, analysts, data product managers, and scientists to improve user experience and increase operational efficiency that grew the business. Before that, he was senior director of engineering at eBay in California and provided leadership in various domains, including data service and solutions, search science, marketing technology, and billing systems. He worked as an architect at IBM prior to eBay, building technology solutions for various clients. Wilson obtained his master’s and bachelor’s degrees in electrical engineering from Zhejiang University in China.