Improving Multilingual LLM Performance with Supervised Fine-Tuning
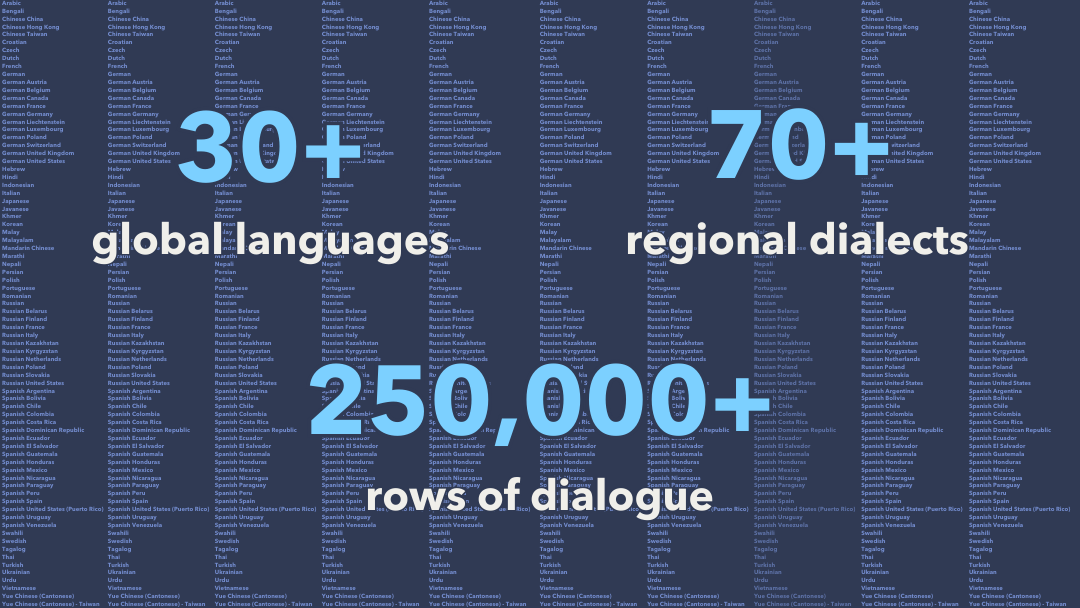
Introduction
Appen supported a global technology company in improving its LLM’s performance across 70+ dialects in 30+ languages by providing LLM training data in the form of structured human feedback. Contributors engaged in multi-turn dialogues, ranking responses from five model variations based on criteria related to response relevance, coherence, accuracy, and fluency. Over 250,000 dialogue rows were collected, refining model outputs for supervised fine-tuning. The project expanded from 10+ dialects in 5+ languages to over 70 dialects, enhancing cultural alignment and language accuracy in model responses.
Goal
The project focused on improving the LLM’s ability to generate high-quality responses across a wide range of dialects – such as Arabic, Chinese, German, Russian, and Spanish – and deliver more accurate, contextually relevant, and fluent outputs tailored to diverse linguistic communities. This required collecting human preference rankings of model outputs and leveraging structured refinement to ensure better cultural and linguistic alignment.
Challenge
The project required high-quality prompts across multiple languages and dialects. Dialogues varied in subject-matter and level of complexity to provide well-rounded AI training data. Furthermore, the evaluation of model responses needed to be localized and factor in unique characteristics of the different dialects and regions. Where model responses showed room for improvement, contributors needed to deliver refined revisions suitable for supervised fine-tuning (SFT).
The scale of this project presented unique challenges, including:
- Recruitment of linguistically diverse contributors: Identifying qualified contributors, particularly for less commonly spoken languages such as Khmer and Marathi.
- Diversity of languages and dialects: Ensuring that prompts and model responses were culturally appropriate and linguistically accurate for each region.
- High-quality evaluation at scale: Conducting dialogue evaluations across multiple complexity levels while maintaining a consistent standard of AI data quality.
- Data suitability for fine-tuning: Ensuring that, when revisions to the model’s outputs were necessary, contributor’s refined responses to meet the quality standards required for SFT.
Solution
To address these challenges, Appen implemented a structured, multi-step approach:
- Expert Contributor RecruitmentAppen sourced native speakers from diverse regional dialects who possessed experience with LLMs and could create culturally relevant and contextually suitable prompts for both monolingual and cross-lingual interactions.
- Structured Preference Ranking ProcessContributors engaged in multi-turn conversations with five different model configurations, ranking responses based on coherence, factual accuracy, fluency, and instruction-following. These rankings provided critical insights into model performance across dialects.
- Supervised Fine-Tuning PreparationThe refined responses from the ranking process were transformed into high-quality training data, ensuring alignment with real-world linguistic and cultural nuances.
- AI Data Platform IntegrationThe project was managed within Appen’s AI data platform (ADAP), enabling efficient workflow execution and quality assurance. Validators and test questions were incorporated to enhance data consistency and accuracy.
Results
The structured ranking and fine-tuning approach significantly improved the client’s LLM performance across multiple dialects, ensuring better cultural alignment and linguistic accuracy. Key outcomes include:
- Over 250,000 rows of dialogue data delivered to date.
- Expansion from an initial 10+ dialects across 5+ languages to over 70 dialects across 30+ languages.
- Improved model accuracy and user satisfaction through enhanced response quality and linguistic diversity.
By leveraging human preference rankings and structured fine-tuning, Appen enabled the client to refine their LLM, making it more responsive to global users and capable of delivering contextually appropriate and accurate responses across a vast linguistic landscape.