Data-Centric AI: The Power of AI Data Quality
Access to accurate, relevant, and unbiased data is critical to the future of AI. This guide outlines the key concepts, benefits, and strategies for implementing data-centric AI.
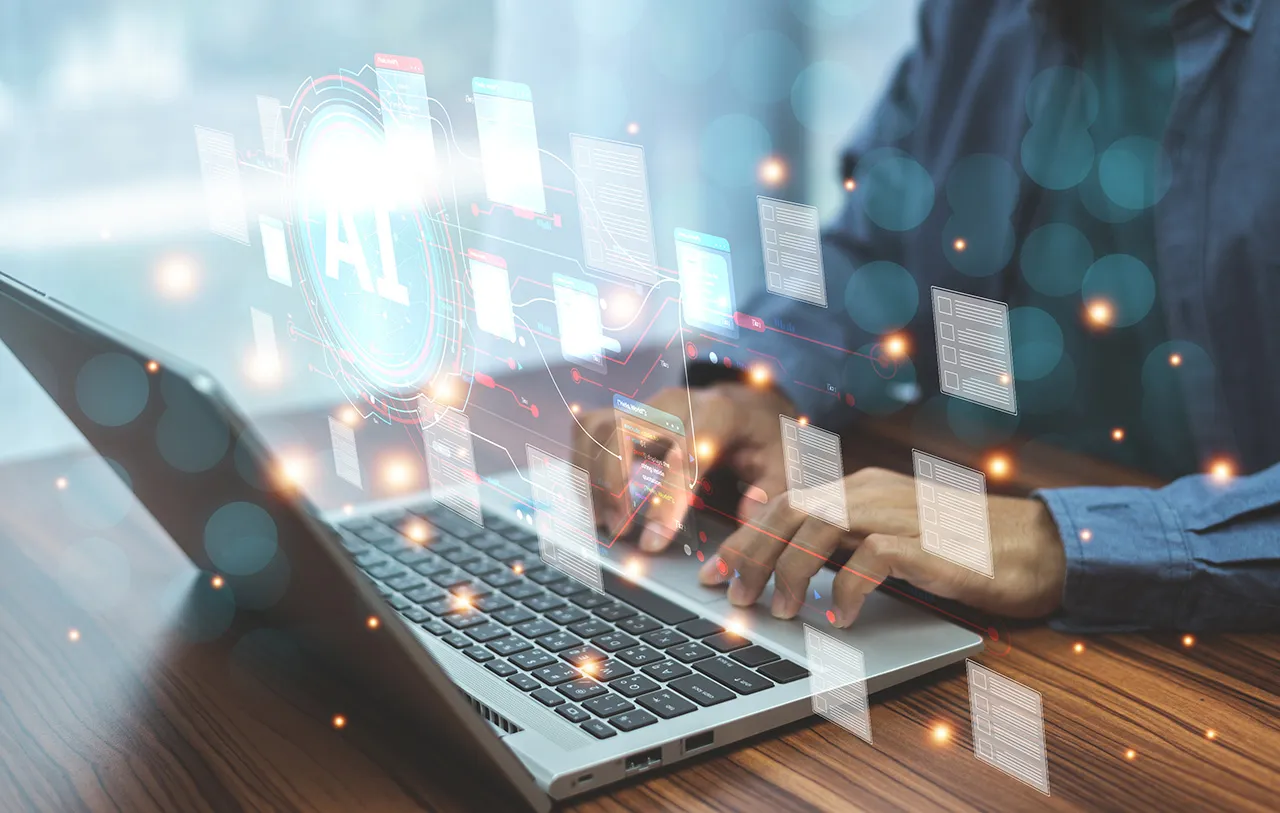
What is Data-Centric AI?
Data-centric artificial intelligence prioritizes data quality in order to develop more efficient and robust AI models. In many cases, a simple model trained on high-quality data can outperform a more complex model trained with noisy or incomplete data. Emphasizing data quality, accuracy, and diversity ensures your model is better equipped to handle real-world scenarios and improves generalization, leading to more reliable outcomes.
Model-Centric vs Data-Centric AI
AI is built upon three foundations: computing, algorithms, and data. GPUs can be purchased easily in most regions and open-source algorithms are readily accessible. This leaves data as the greatest opportunity for differentiation in the market.
While model-centric AI emphasizes algorithmic refinement, data-centric AI shifts the focus to optimizing data quality. By training AI models on data that reflects the complexity of real-world environments, data-centric AI enhances the model’s ability to make more informed, reliable, and ethical decisions while also reducing the need for constant algorithmic refinement.
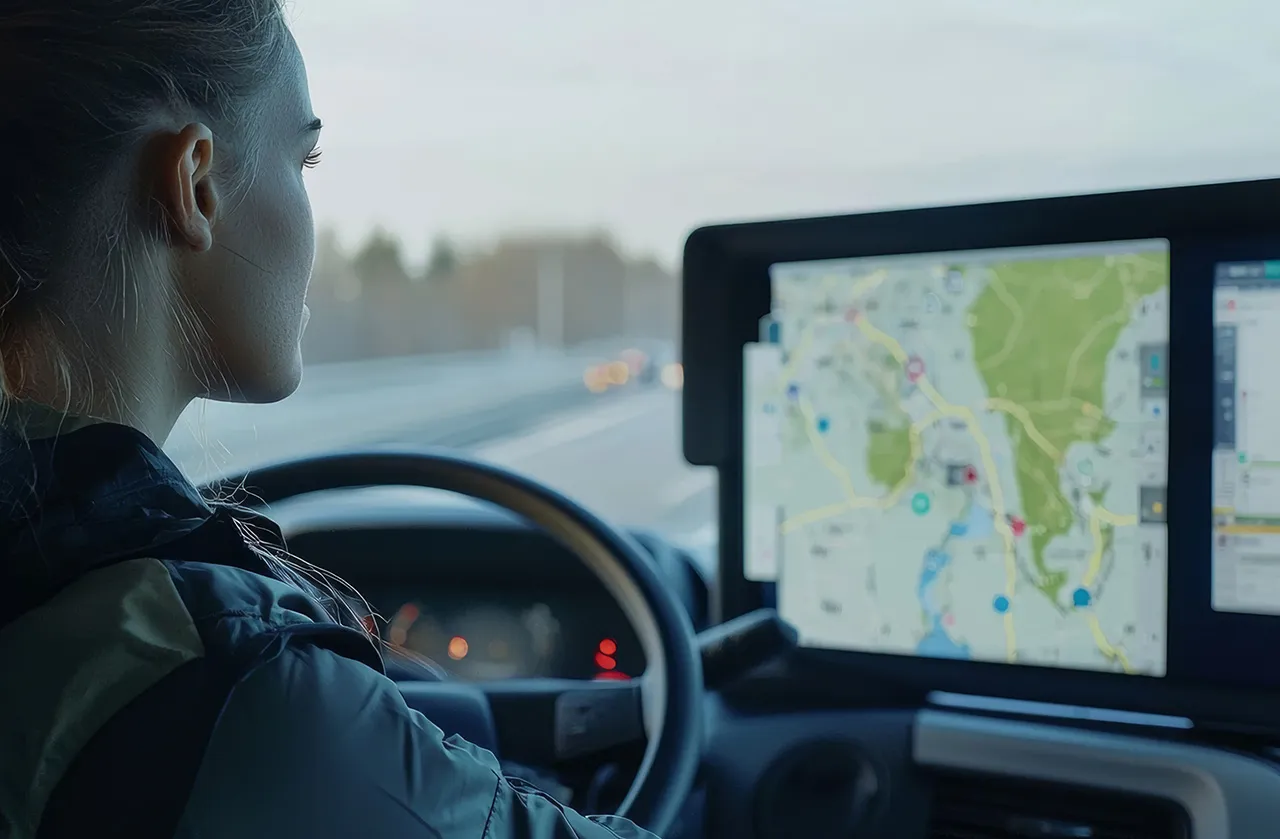
Why Data-Centric AI Matters
High-quality data is critical to AI success. Custom data improves model performance, enabling small models to perform equal to larger ones by leveraging specific and high-quality training data, while models built on poor or biased data yield inaccurate predictions and increase the risk of AI hallucinations. Data-centric AI ensures that AI models are trained on clean, representative data, leading to more accurate and ethical results.
Benefits of Data-Centric AI
Enhanced Performance
Prioritizing data quality leads to more accurate model output in key tasks such as training an OCR algorithm on relevant documents.
Faster Development
High-quality data reduces the need for retraining and model updates and enables smaller models to perform equal to larger ones.
Cost Efficiency
Reliable data allows for simpler model development, lowering resource consumption during model building and refinement.
Fairness and Ethics
Reducing bias in datasets leads to more ethical AI, which is crucial for maintaining trust and safety in AI systems.
Challenges and Solutions of Data-Centric AI
Key Components of Data-Centric AI
Optimize your model by ensuring your data is accurate, consistent, and reflective of real-world scenarios.
Data Quality Management
AI data quality best practices include:
- Human-Generated Data: Trust human expertise for sensitive and nuanced tasks for greater accuracy than web-scraped or synthetic data.
- Diverse Data Sources: Diverse datasets ensure models are more adaptable to real-world scenarios.
- Ethical Sourcing: Source data responsibly to reduce biases and ensure compliance with regulations.
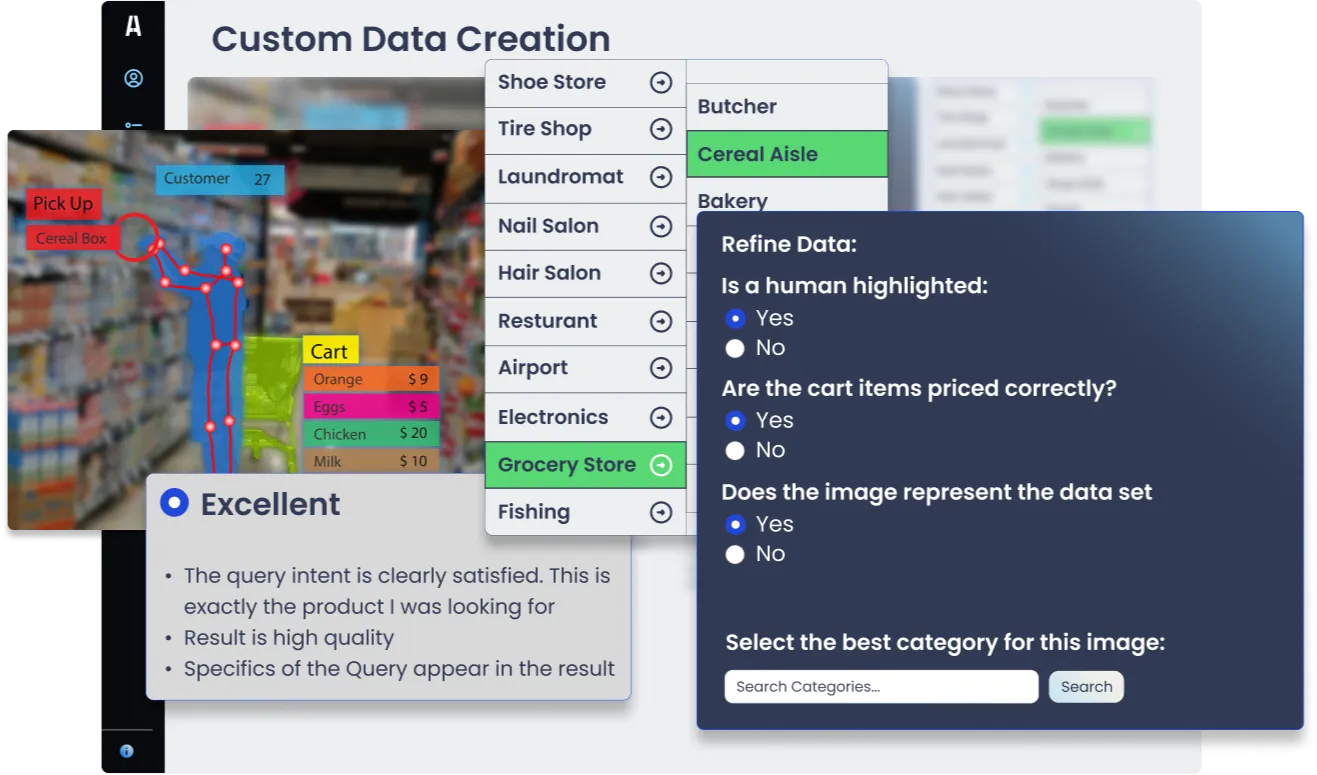
Data Annotation
High-quality data annotation is essential for supervised learning models. Key practices include:
- Human-in-the-Loop: Integrate human oversight with AI for more efficient and cost-effective data annotation.
- Active Learning: Accelerate the data annotation process with machine learning and more efficiently clean and label data.
- Consistent Results: Clear data annotation guidelines are essential to maintaining consistency across large datasets.
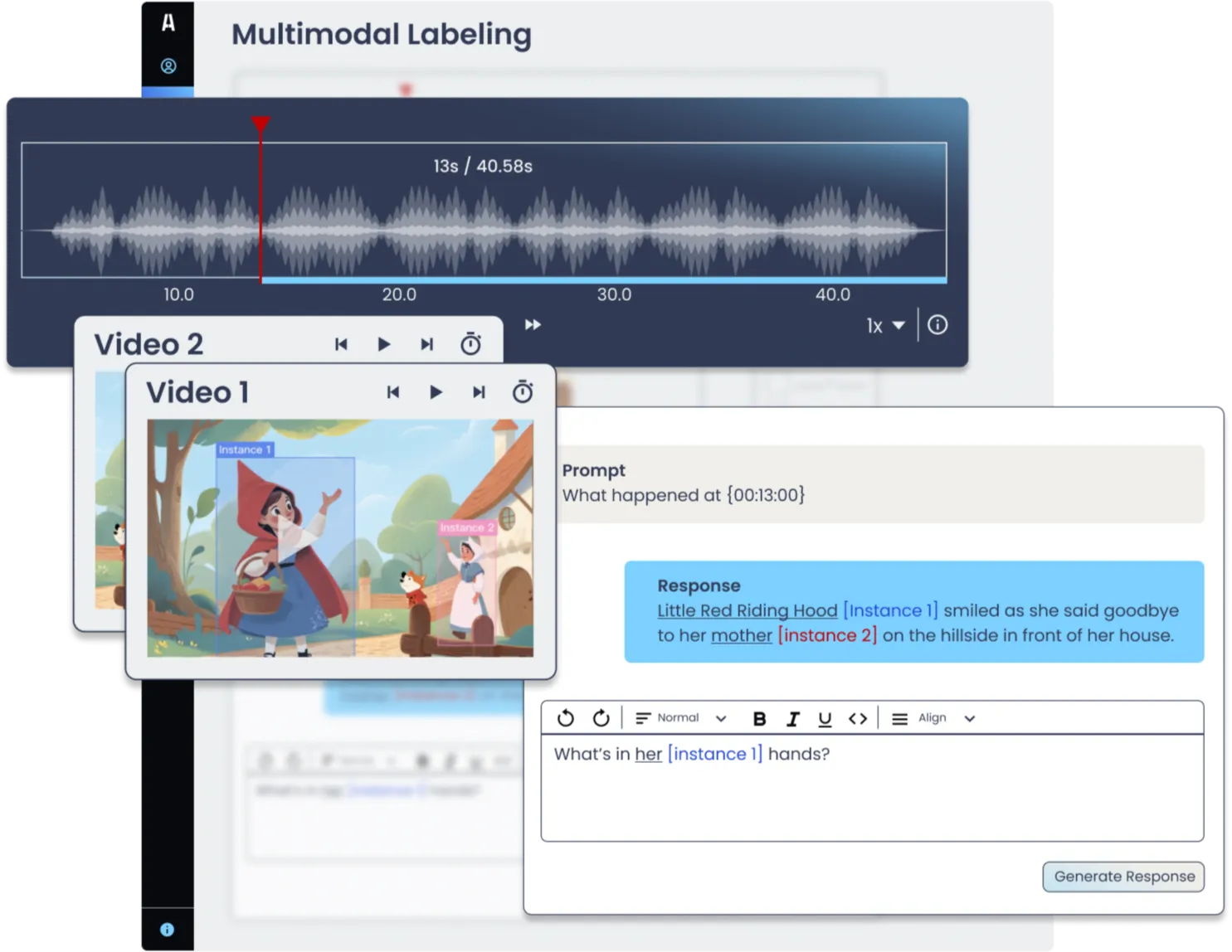
Handling Data Bias
Bias in datasets can lead to unfair or skewed AI outcomes. To combat this:
- Identifying Bias: Regularly review datasets to identify and address underrepresented or overrepresented groups.
- Correcting Bias: Apply techniques such as red teaming and oversampling to balance biased datasets.
- Continuous Monitoring: Periodically update and audit datasets to maintain fairness over time.
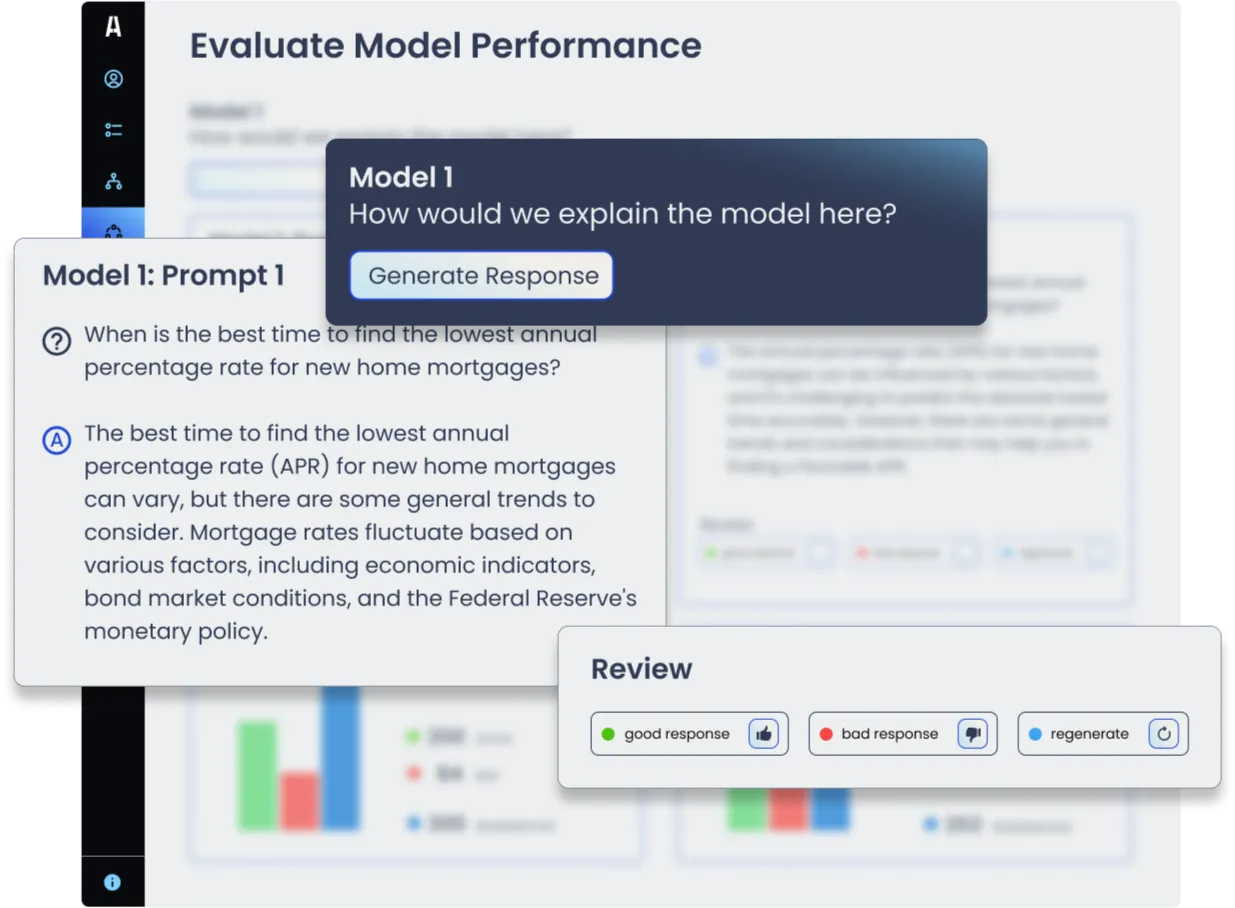
How to Implement Data-Centric AI
Shifting to a data-centric AI approach can significantly enhance AI development. Follow these steps:
Assess Your Data Needs
Evaluate your goals and existing data to identify gaps. Appen’s AI experts can help you define your project requirements and job instructions to guide data collection and annotation.
Define Data Quality Standards
Establish clear benchmarks for your data to ensure a high standard of quality control. Appen can help you implement data quality best practices that include accuracy, diversity, and annotation consistency.
Clean and Curate Your Data
Leverage data management tools to deduplicate, reduce noise, and detect outliers in your dataset. Streamline data collection, cleaning, and annotation all in one place with Appen’s AI Data Platform.
Continuously Monitor and Improve
Data is not static. Regularly monitor and update your data pipelines to ensure they meet evolving standards. Appen’s platform enables you to track changes and keep your datasets up to date.
Human Expertise is Essential to High-Quality Data
While AI and automation enhance efficiency, human expertise is crucial for ensuring accuracy. Many tasks require nuanced understanding of language in context, such as sentiment analysis and machine translation.
Including subject matter experts in your data pipeline allows organizations to capture subtleties that AI models might overlook, reducing error rates and improving overall data quality. Experts also play a critical role in monitoring datasets for bias, ensuring fairness and ethical decision-making. By integrating human expertise in data collection, annotation, and model evaluation, organizations can build more reliable and adaptable AI models capable of handling real-world challenges.
Watch the Webinar: Optimize LLMs with Human-AI Collaboration

Appen’s Data-Centric
AI Solutions
Appen is a global leader in AI and machine learning data solutions with over 25 years of experience. We provide end-to-end services that ensure your AI projects are powered by the highest-quality data:
Data Collection
Leverage our crowd of over 1 million contributors worldwide to gather diverse and reliable data.
Data Annotation
Appen offers advanced annotation tools and expert crowdsourcing to ensure precise datasets for your AI models.
Model Evaluation
Optimize of your AI models, ensuring they remain accurate and adaptable, with techniques like red teaming and retrieval-augmented generation.
Ready to Shift to Data-Centric AI?
Take the next step in your data-centric AI journey with Appen’s data solutions. Contact us today to discover how we can help you collect, annotate, and refine the data your AI models need to succeed.