What You Need to Know About Quality Assurance for Your AI Models
Launching an artificial intelligence (AI) model that’s accurate, reliable, and unbiased is undeniably a challenge. Organizations that manage to achieve success in AI initiatives are likely aware that the quality assurance (QA) process is very different with AI than traditional QA processes.Quality assurance plays a critical role in the accuracy of an AI model and shouldn’t be overlooked. Any company hoping to deploy effective AI must build in QA checks throughout their model’s lifecycle.We often discuss the five phases of building world-class AI, which include:
- Pilot
- Data Annotation
- Test & Validate
- Scaled Deployment to Production
- Retraining
During the five-phase lifecycle of an AI project, a QA team should perform various checks and reviews. There are three ways quality assurance processes should be applied, depending on what phase you are in.
Phases 1 and 2: Pilot and Data Annotation
This is when businesses should be identifying the problem they’re going to solve and gathering the data. QA confirms the data used for model training is of sufficient quality.
Phases 3 and 4: Test & Validate and Scaling
During these phases, the model is built and is being tested and tuned as it scales to a wider and wider audience. QA is vital during these phases as it verifies the built model that goes live is of sufficient quality - especially as the model runs off real data, not test data.
Phases 5: Retrain
Regular retraining is critical for almost every AI model. QA confirms the model continues to deliver sufficient quality when running and offers the opportunity to continue to improve accuracy.Several QA steps require a check comparing a metric of your data or model to a predefined value or threshold. Others are analyses or reviews, requiring time, manual effort, domain knowledge, and common sense. In any case, building in QA checks and balances is an integral component of deploying successful AI.
Quality Assurance and Training Data
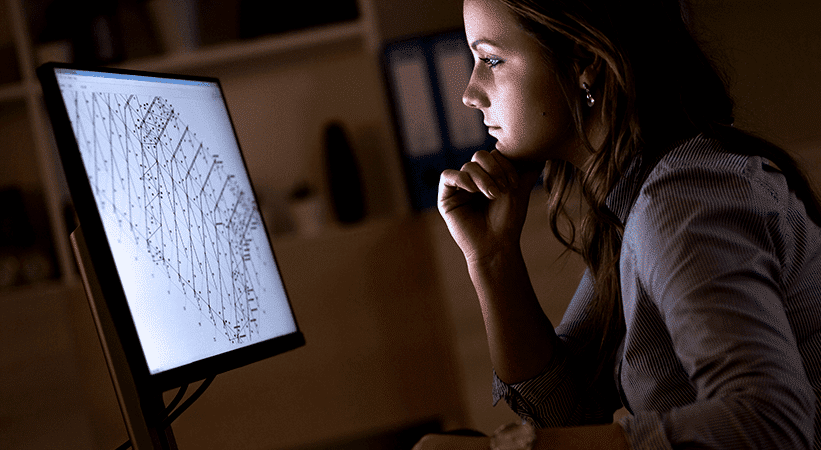
Where QA plays perhaps the most vital role is in monitoring the quality of training data. Training data is the core ingredient in making AI work, as a model is only as good as the data it was trained on. Developers use training data to teach AI models to process and make inferences in ways that satisfy the hyperparameter configuration. In other words, AI models are accurate, reliable, and unbiased only if the data they were trained on is also all of those things.To ensure training data fits the model, the data itself has to be tested for quality, completeness, reliability, and validity. This includes identifying and removing any sort of human bias. In real-world scenarios, the data that an AI model processes may be a stretch from what it was trained on; training data must then be diverse enough to prepare the model for real-life applications.QA testing of training data is done to ensure that the parameters used to configure the AI model can perform at capacity and meet the desired performance standards. This is done through a series of validation processes: feeding the model with training data and valuing the outcomes (inferences) it produces. If the outcomes aren’t up to desired standards, then developers rebuild the model and process the training data again.QA testing isn’t merely a procedure that AI developers must complete, but an instrumental process in ensuring that intelligent machines can effectively drive operations to new highs and maximize efficiency.
How We Ensure Quality and Accuracy
At Appen, we provide our customers with enhanced QA processes throughout your model build. We have built-in quality features including test questions, redundancy, and the ability to target specific crowd types to ensure quality is consistently monitored and enforced in your jobs. We also have dedicated customer success resources to help you with onboarding, job design, monitoring, and optimization.We offer a range of data annotation options (including the option to supply your own internal crowd) to suit your AI model needs with over 180 different languages and dialects we support. With our crowd residing in the same ecosystem, we can apply consistent quality controls across the entire annotation pipeline. We do this through three levers:Test QuestionsOur patented framework utilizes pre-answered rows of your data to qualify high-performing contributors, remove low-performing ones, and continually train contributors to improve their understanding of the task.RedundancyWe have multiple trusted contributors annotate each row of your data. In doing so, we can ensure agreement is reached and any individual bias is controlled.Contributor LevelsWe keep an audit trail of every contributor and categorize them into three levels based on performance and experience on the platform. Level 1 can be used to optimize throughput, while Level 3 ensures only our most experienced and highest performers will work on your task.We recognize the importance of QA in all facets of the AI lifecycle, and our team of experts is poised to provide needed expertise throughout. Accuracy, reliability, and bias reduction is possible through comprehensive quality assurance efforts and having a trusted data partner who performs quality controls can help you achieve these results in a way that lets you scale quickly.