Machine learning requires high volumes of data for training, validation, and testing. A machine learning model learns to find patterns in the input that is fed to it. This input is referred to as training data. As you train your solution to form relationships between variables, it’s important to have the right data, structured in the right format, covering all the variation your solution will encounter in the real world.So how do you get the large volume of structured data you need? Human-annotated data is the key to successful machine learning. Humans are simply better than computers at managing subjectivity, understanding intent, and coping with ambiguity. For example, when determining whether a search engine result is relevant, input from many people is needed for consensus. When training a computer vision or pattern recognition solution, humans are needed to identify and annotate specific data, such as outlining all the pixels containing trees or traffic signs in an image. Using this structured data, machines can learn to recognize these relationships in testing and production.Most AI applications require models to be continuously trained to adjust to changing conditions. Most use cases for machine learning require ongoing data acquisition — with some requiring model refreshes monthly or daily.
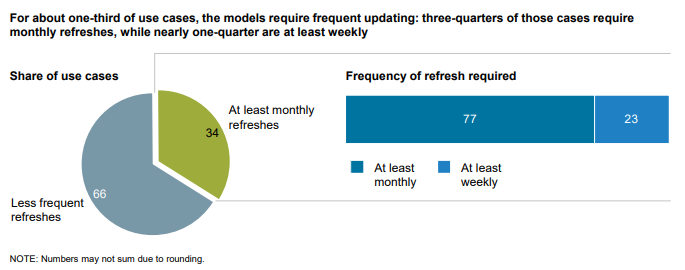
Source: McKinsey Global Institute analysisFor over 20 years, Appen has helped clients optimize their machine learning models by collecting and annotating data across text, speech, audio, image, and video formats. Here’s how three leading global technology companies have gained more value from their machine learning investments with high-quality training datasets from Appen:
Use Case 1: Improving Search Quality for Microsoft Bing in Multiple Markets
Microsoft’s Bing search engine required large-scale datasets to continuously improve the quality of its search results – and the results needed to be culturally relevant for the global markets they served. Appen delivered results that surpassed expectations. Beyond delivering project and program management, Appen provided the ability to grow rapidly in new markets with high-quality data sets. (Read the full case study here)
Use Case 2: Improving Relevance of Social Media Content for a Leading Global Platform
One of the world’s top social media platforms needed to improve the personalization of its news feed due to user feedback. To facilitate that, the company’s algorithm required an accurate representation of their diverse user base. Starting with a 4-week pilot project in which 500 annotators rated news feed items on relevance and importance of the content, the partnership expanded to become an ongoing, years-long program involving thousands of annotators. Not only did their news feed become much more personalized, but the client can now apply similar processes to address other areas of their site. (Read the full case study here)
Use Case 3: Improving Listings with Local Search Content Evaluation for a Global Search Engine
Users of a leading multilingual search engine provider rely on its data to find local business information, including addresses, phone numbers, hours of operation, maps, and directions. The search engine relied on algorithms supported by an inhouse team to serve the most relevant and accurate results to its users. The firm’s existing process for adding and updating local business information was unable to keep up with the increased presence of businesses on the internet. Failure to keep local listings up-to-date could result in users losing confidence in the search engine’s data and turning to its competition for future queries. Verifying that local listings were being returned accurately was of critical importance to retaining the search engine’s local site traffic. What started with 10 annotators in one market grew to over 440 annotators in 31 markets. To improve local search listings and enhance user experience, Appen annotators verified and corrected data for more than 750,000 of the client’s business listings. (Read the full case study here)— Interested in how Appen’s human-annotated data can help your company? Connect with one of our experts.