Top Eight Ways to Overcome and Prevent AI Bias
Algorithmic bias in AI is a pervasive problem. You can likely recall biased algorithm examples in the news, such as speech recognition not being able to identify the pronoun “hers” but being able to identify “his” or face recognition software being less likely to recognize people of color. While entirely eliminating bias in AI is not possible, it’s essential to know not only how to reduce bias in AI, but actively work to prevent it. Knowing how to mitigate bias in AI systems stems from understanding the training data sets that are used to generate and evolve models.In our 2020 State of AI and Machine Learning Report, only 15% of companies reported data diversity, bias reduction, and global scale for their AI as “not important.” While that’s great, only 24% reported unbiased, diverse, global AI as mission-critical. This means that numerous companies still need to make a true commitment to overcoming bias in AI, which is not only indicative of success, but critical in today’s context. Because AI algorithms are meant to intervene where human biases exist, they’re often thought to be unbiased. It’s important to remember that these machine learning models are written by people and trained on socially generated data. This poses the challenge and risk of introducing and amplifying existing human biases into models, preventing AI from truly working for everyone.Responsible and successful companies must know how to reduce bias in AI, and proactively turn to their training data to do it. To minimize bias, monitor for outliers by applying statistics and data exploration. At a basic level, AI bias is reduced and prevented by comparing and validating different samples of training data for representativeness. Without this bias management, any AI initiative will ultimately fall apart.Here are eight ways you can prevent AI bias from creeping into your models.
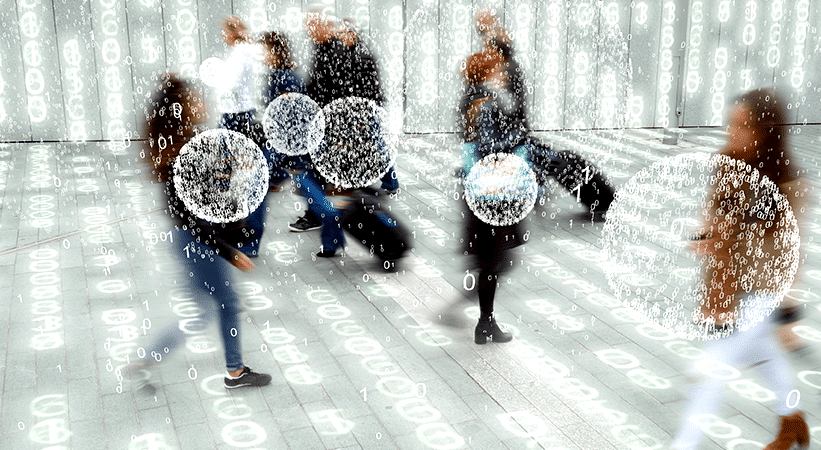
Eight Steps on How to Reduce Bias in AI
- Define and narrow the business problem you’re solvingTrying to solve for too many scenarios often means you’ll need a ton of labels across an unmanageable number of classes. Narrowly defining a problem, to start, will help you make sure your model is performing well for the exact reason you’ve built it.
- Structure data gathering that allows for different opinionsThere are often multiple valid opinions or labels for a single data point. Gathering those opinions and accounting for legitimate, often subjective, disagreements will make your model more flexible
- Understand your training dataBoth academic and commercial datasets can have classes and labels that introduce bias into your algorithms. The more you understand and own your data, the less likely you are to be surprised by objectionable labels.
- Gather a diverse ML team that asks diverse questionsWe all bring different experiences and ideas to the workplace. People from diverse backgrounds –race, gender, age, experience, culture, etc. – will inherently ask different questions and interact with your model in different ways. That can help you catch problems before your model is in production.
- Think about all of your end-usersLikewise, understand that your end-users won’t simply be like you or your team. Be empathetic. Avoid AI bias by learning to anticipate how people who aren’t like you will interact with your technology and what problems might arise in their doing so.
- Annotate with diversityThe more spread out the pool of human annotators, the more diverse your viewpoints. That can really help reduce bias both at the initial launch and as you continue to retrain your models.
- Test and deploy with feedback in mindModels are rarely static for their entire lifetime. A common, but major, mistake is deploying your model without a way for end-users to give you feedback on how the model is applying in the real world. Opening up a discussion and forum for feedback will continue to ensure your model is maintaining optimal performance levels for everyone.
- Have a concrete plan to improve your model with that feedbackYou’ll want to continually review your model using not just customer feedback, but also independent people auditing for changes, edge cases, instances of bias you might’ve missed, and more. Make sure you get feedback from your model and give it feedback of your own to improve its performance, constantly iterating toward higher accuracy.
How to Reduce Bias in AI With Appen
At Appen, we have spent the last 20+ years annotating data, leveraging our diverse crowd to help ensure you can confidently deploy your AI models. We can help you avoid AI bias that lands you on the list of biased algorithm examples by not only supplying you with a platform with over one million crowd members from 130 countries, but we can also set you up with our managed service team of experts to produce the best training data for your AI models.